From pricing to rating structured credit products
and vice-versa
Quentin Lintzer Université Paris VI - Pierre &
Marie Curie
Thesis submitted for the degree of: Master M2 in Probability
Theory and Applications
· September 2007 ·
Abstract
Credit risk area is one of the most rapidly developing areas
in finance. A wide range of synthetic structured credit products builds on
liquid credit instruments such as Credit Default Swaps, credit indices or
Credit Synthetic Obligations (CSO) referenced on the latter. In this document,
we first recall the principles of CSO pricing in a one factor gaussian copula
model and outline two numerical procedures aimed at mapping joint loss
distributions. We also formalize the theoretical modelling framework of
Moody's, a rating agency, for rating Constant Proportion Debt Obligation (CPDO)
and Constant Proportion Portfolio Insurance (CPPI) products. We then present
our conclusions regarding an innovative Dynamic Proportion Portfolio Insurance
(DPPI) product and raise some risk management issues.
Contents
1
|
Structured credit products: a business review
1.1 Introduction
1.2 Elementary building blocks
|
4 4 4
|
|
|
1.2.1
|
Credit Default Swaps
|
4
|
|
|
1.2.2
|
Credit indices
|
6
|
|
|
1.2.3
|
Collateralized Synthetic Obligations
|
6
|
|
|
1.2.4
|
Structured Non-Correlation Products
|
9
|
2
|
Modelling and pricing CSO tranches
|
12
|
|
2.1
|
Modelling a CSO tranche payoff
|
12
|
|
2.2
|
Default and premium legs of a CSO tranche
|
12
|
|
|
2.2.1
|
Default leg
|
13
|
|
|
2.2.2
|
Premium leg
|
13
|
|
|
2.2.3
|
Fair premium
|
13
|
|
2.3
|
The semi-analytic approach: one factor Gaussian Copula model . .
.
|
14
|
|
|
2.3.1
|
Copula functions: basic properties
|
14
|
|
|
2.3.2
|
The one factor gaussian copula model
|
14
|
|
2.4
|
Back to CSO tranche pricing: computing the expected portfolio
loss
|
16
|
|
|
2.4.1
|
Monte-Carlo simulations
|
16
|
|
|
2.4.2
|
Evaluating the loss characteristic function
|
16
|
|
|
2.4.3
|
Andersen's recursive formula
|
17
|
3 Modelling and Rating Dynamic Proportion Portfolio Insurance
prod-
ucts 19
3.1
|
Moody's approach to rating CPDO and
|
|
|
CPPI/DPPI products
|
19
|
|
3.1.1
|
Historical vs risk-neutral probability measures
|
19
|
|
3.1.2
|
Moody's Metric and coherent risk measures
|
20
|
3.2
|
Modelling risk factors
|
23
|
|
3.2.1
|
Credit spread processes influenced by defaults and ratings .
.
|
23
|
|
3.2.2
|
Rating migrations and default events
|
24
|
|
3.2.3
|
Interest rates process and other parametres
|
26
|
3.3
|
Portfolio investment rules
|
27
|
|
3.3.1
|
Dynamic leverage function
|
27
|
|
3.3.2
|
Deferred coupons
|
29
|
|
3.3.3
|
Other key structural features
|
30
|
3.4
|
A study of the DPPI's sensitivities
|
30
|
|
3.4.1
|
Tailor-made structural features to achieve target rating. . .
.
|
31
|
|
3.4.2
|
Hypothetical stress-scenarios
|
35
|
3.5 DPPI: any hidden pricing issue? 36
3.5.1 From the investor's perspective 36
3.5.2 From the investment bank's perspective 36
Conclusion 37
Appendix 38
Bibliography 42
List of Figures
1.1
|
Cash flows of a Credit Default Swap with physical delivery
|
5
|
1.2
|
Structuring of a single-tranch CSO
|
7
|
1.3
|
Structuring of a first-generation CPDO, referencing credit
indices . .
|
9
|
3.1
|
Moody's rating conversion table
|
21
|
3.2
|
Moody's idealized EL values by rating category and tenor
|
22
|
3.3
|
DPPI base-case loss distribution conditional on the structure
not cash-
|
|
|
ingin
|
31
|
3.4
|
Distribution of cash-in times
|
31
|
3.5
|
Estimated expected loss as a function of S.
|
32
|
3.6
|
Estimated expected loss as a function of è (2000
simulations per
|
|
|
coupon level)
|
33
|
3.7
|
Loss and Moody's Metric as a function of OL and TM, 1000
simula-
|
|
|
tions per couple of parametres
|
34
|
8
|
Parametres of Moody's CDS spread processes
|
39
|
9
|
Correlation matrix of Moody's CDS spread processes
|
39
|
10
|
Moody's 10Y corporate rating transition matrix
|
39
|
11
|
DPPI optimized structural features
|
40
|
12
|
DPPI reference portfolio
|
41
|
Chapter 1
Structured credit products: a
business review
1.1 Introduction
Credit derivatives markets have been consistently among the
fastest growing areas of capital markets in recent years: 2006 year-end ISDA
survey shows outstanding notionnal of 34,000 USD Bio for all credit derivatives
contracts, up from 8,000 USD Bio in 2004. Such a growth was fueled by the
appetite of various types of investors for credit risk and relied upon the
ability of investment banks to repackage credit risk into synthetic structured
products.
Before going into the details of modeling and pricing such
credit derivatives, we shall describe the principles of the main products that
can then be used as building blocks for more sophisticated ones. They share the
same underlying risk, that is the credit risk of one or several reference
entities, whether it be a corporate company, a financial institution or even a
soveriegn entity:
· Single-name Credit Default Swaps (CDS) are to credit
derivatives markets what single-name equity stocks are to equity derivatives
markets;
· Collateralized Synthetic Obligations (CSO) aim at
tranching credit risk on an entire portfolio of reference entities, hence
creating correlation risk;
· Constant Proportion Dynamic Portoflio Obligations
(CPDO) and Constant Proportion Portfolio Insurance (CPPI) products allow
investors to take credit risk on diversified portfolios of single names while
avoiding first-order correlation risk;
· Vanilla options on credit indices started trading as
liquidity in underlying CDS contracts and standardized CSO tranches was
increasing.
1.2 Elementary building blocks
1.2.1 Credit Default Swaps
A Credit Default Swap (CDS) is a contract whereby counterpart
A (the «protection seller») receives a periodic premium from
counterpart B (the «protection buyer») and agrees to protect the
latter against the default of entity C (the «reference entity»).
In the event of default, counterpart A would pay counterpart
B the notional amount of a reference obligation (which could be a bond or a
loan) issued by entity C and receive the reference obligation.
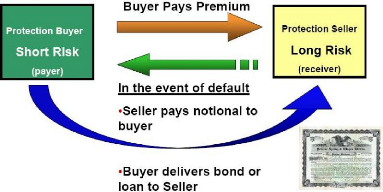
Figure 1.1: Cash flows of a Credit Default Swap with physical
delivery
CDS are quoted in terms of spread (measured in basis points)
over an Inter Bank Offered Rate (EURIBOR or LIBOR depending on the currency).
Assuming a constant recovery rate R for the underlying obligation, we can
easily express this spread as a function of the survival cumulative
distribution function S0(.) of the reference entity defined as follows under
the risk neutral probability measure Q. Let r be the continuous random variable
modelling the instant of default:
?t ? R+,S0(t) = Q(r = t)
By construction, for a given notional amount N, a fixed
recovery rate R, a contract maturity of Tn and risk-free bond prices
B(0, Ti), i ? {1, .., n}, the market spread at inception of a given CDS is
determined such that the present value of the premium leg (the «fixed
leg») equals that of the default leg (the «variable» leg):
Xn B(0, Ti) · EQ [N(1 -
R)1{Ti-1=ô=Ti} ~
i=1
Xn B(0,Ti) · N(1 - R) · [S0(Ti-1) -
S0(Ti)]
i=1
[ Xn ]
= EQ B(0, Ti)sN1{ô=Ti}
i=1
Xn B(0, Ti) · sN · (Ti - Ti-1) ·
S0(Ti)
i=1
As a result, the CDS spread s is given by the following formula,
where D0(Ti) := B(0, Ti)S0(Ti) denotes the risky bond price:
s = (1 - R) ·
|
>n (S0(Ti_1) )
i=1 D(0, Ti) S0(Ti) - 1
|
(1.1)
|
|
|
1.2.2 Credit indices
Credit indices are convenient proxys for taking credit
exposure on diversified portfolios of single names. They can be sorted by
geographical criteria (Europe, Asia, US, Japan, Emerging markets), by
industrial sector (e.g. Financials) or by debt riskiness criteria (investment
grade, high yield). Among all indices, one shall bear in mind the ones detailed
hereafter:
Index name
|
Debt riskiness
|
Characteristics
|
CDX.NA.IG
|
Investment grade
|
Diversified portfolio of 125 North American liquid corporate
credits
|
CDX.NA.HY
|
High Yield
|
Broad-based portfolio of 100 high yield credits, i.e.
sub-investment grade
|
ITRAXX IG
|
Investment grade
|
Diversified portfolio of 125 European liquid corporate
credits
|
ITRAXX XOVER
|
High Yield
|
Diversified portfolio of 125 European
high yield credits, i.e. sub-investment grade
|
|
Similarly to single-name CDS, they are quoted in terms of
spread (measured in basis points) over LIBOR/EURIBOR rate. Given that all
single-name components are equally weighted in credit indices, an event of
default on a single name triggers a proportionate reduction in the notional
amount of the index and a lump sum payment from the protection seller to the
protection buyer.
1.2.3 Collateralized Synthetic Obligations Basic
principles
Collateralized Synthetic Obligations (CSO) are securities
issued by a Special Purpose Vehicle (SPV) and backed by a portfolio of credit
protection-selling positions taken through several (usually over 100) CDS. The
liabilities of the SPV get sliced into several CSO tranches that get hit
sequentially in case one or more reference entities within the underlying CDS
portfolio default. As a result, the fair premium of any tranche, usually
expressed as a spread over 3-month LIBOR/EURIBOR rate, eventually depends on
the joint-loss distribution of the underlying CDS portfolio.
CSO tranches can be defined by their attachment and detachment
points:
· The attachment point l of the tranche is expressed as
a percentage of the investment notional. It is the portfolio loss lower
threshold above which the tranche's principal gets hit if one or several
reference entities default within the portfolio.
· The detachment point u > l of the tranche is
expressed as a percentage of the investment notional. It is the portfolio loss
upper threshold above which the tranche's principal gets wiped out after one or
several events of default.
CSO tranches are labelled upon their seniority in the capital
structure:
· The equity tranche has the lowest attachment point
of the structure - 0% - and usually a detachment point below 3%. Hence it is
the riskiest tranche of the structure.
· The super senior tranche has the highest attachment point
of the structure - usually around 22% - and a detachment point of 100%.
· Mezzanine tranches have an attachment point above the
equity's detachment point and a detachment point below the senior's attachment
point.
· Senior tranches have an attachment point above the
mezzanine's detachment point and a detachment point below the supersenior's
attachment point.
Unlike cash Collateralized Debt Obligations (CDO), CSOs are
not backed by a portfolio of physical bonds or loans but by a portfolio of CDS
contracts. This latter feature allows much more flexibility in structuring
tailor-made securities than cash-based CDOs:
· Physical bonds or loans only exist in limited quantity,
whereas CDS contracts can be created as long as two counterparties agree to
trade with each other;
· Whenever a cash-CDO is structured, all tranches must
be sold to the investors, i.e. the deal must be fully syndicated, unless the
CDO-arranging bank wants to keep some risk in it books, whereas CSO tranches
can be structured independently because their payoffs can be replicated through
model-based offsetting CDS positions.
· Transaction follow-up duties are heavier for cash-CDOs
than for CSOs: for instance, loans can be subject to contingent early
repayments.
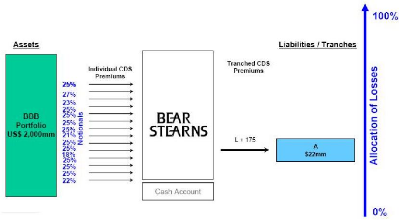
Figure 1.2: Structuring of a single-tranch CSO
CSO tranches on credit indices
As mentioned earlier, the price of a CSO tranche, which is
defined by its attachment and detachment points within the capital structure,
is a function of the joint-loss distribution of an underlying reference CDS
portfolio. This joint-loss distribution function can be modeled in terms of two
sets of parametres:
· single-name CDS spreads can be seen as proxies for
valuing the default risk of each reference entity;
· cross-asset default risk dependency parametres, i.e.
«correlation», that aim at describing the joint default-behaviour of
a portfolio of reference entities.
The rationale for setting up a liquid market for tranches
with standardized characteristics (attachment and detachment points) and
referencing standard CDS portfolios (typically ITRAXX IG or
CDX.NA.IG for 3,5,7 and 10 year-maturities)
was to make correlation tradable, thereby allowing flexible correlation hedging
for structured credit products.
Given that this market for standardized CSO tranches on
credit indices aims at pricing correlation only and not default risk on any
single name, tranches are quoted in terms of credit spread (measrued in basis
points) on a Delta-Exchange basis: in other words, tranches and offsetting CDS
positions are traded at the same time so that the resulting exposure of the
investor is only to correlation and not to single-name first-order spread risk.
Unlike other tranches which are quoted as a full running spread, the equity
tranche (0%-3%), which is the riskiest slice of the capital structure, is
quoted on a running basis assuming the protection seller on this tranche
receives a 5% upfront premium.
Bespoke Collateralized Synthetic Obligations
Bespoke CSOs are tailor-made versions of CSO tranches on
credit indices: the underlying CDS portfolio can be customized, as well as the
characteristics of the tranche. This range of products offers more flexibility
than standard tranches, for it allows the investor to choose his own credit
risk profile by playing with the shape of the joint-loss distribution function
(bespoke CDS portfolio) and choosing the attachment/detachment points that suit
his aversion to risk.
In addition to tailoring the initial underlying CDS portfolio
to the investor's needs, investment banks usually propose managed versions of
bespoke CSOs that allow the underlying CDS portfolio to be revised later on in
the transaction's life: the arranging bank appoints an external credit risk
manager whose role is to manage actively the underlying CDS portfolio by making
substitutions and weight adjustments among referenced single names.
Options on credit indices and tranches
As the liquidity of credit indices keeps improving, bid-offer
spreads decrease and investment banks start proposing swaptions on major credit
indices (ITRAXX XOVER, ITRAXX IG, CDX NA IG,...) on standard maturities (roll
dates, i.e. 20-Mar, 20- Jun, 20-Sep, 20-Dec) and tenors (3Y,5Y,7Y).
1.2.4 Structured Non-Correlation Products
Unlike CSO tranches, the value of which relies heavily on
correlation assumptions linking default probabilities on single names, a range
of «correlation-free» structured credit products has emerged since
2004. Constant Proportion Portfolio Insurance (CPPI) and Constant Proportion
Debt Obligation (CPDO) products reference CDS portfolios, but their joint-loss
distribution is not tranched among investors.
Constant Proportion Debt Obligation: «the more you lose,
the more you bet»
First introduced by ABN-Amro in S2 2006, a Constant
Proportion Debt Obligation is a security whose principal and coupons are rated
AAA by rating agencies such as S&P and Moody's and that pays to the
noteholder quarterly EURIBOR/LIBOR coupons plus a spread around 100-200 bps
depending on issuing market conditions. Such a return is achieved by selling
credit protection on credit indices or on a portfolio of single-name CDS in an
amount that is adjusted dynamically throughout the transaction's lifetime: this
dynamic «leverage» function can reach as much as 15 times the initial
notional.
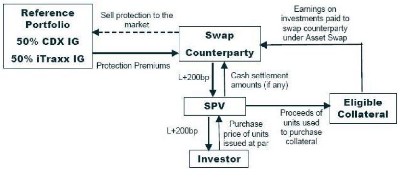
Figure 1.3: Structuring of a first-generation CPDO, referencing
credit indices
Let us define the following variables at time t in order to
summarize the few investment guidelines that rule the CPDO's behaviour:
· A = Notional of the security;
· NPV = Net Present Value of the security;
· MtM = Marked-to-Market value of all long positions on
credit indices and/or single-name CDS;
· Collat = Value of the assets collateralized in the
transaction to serve the EURIBOR/LIBOR component of the coupon;
· CA = Balance of the Cash Account of the structure; in
particular, can be affected by default losses;
· TRV = Target Redemption Value of the security;
· PVNotional = Present Value of the security's Notional as
discounted per the risk-free discount curve;
· PVCoupons = Present Value of the future coupons of the
security discounted as per the risk-free discount curve;
· PVFees = Present Value of the future running fees to be
paid by the noteholder and discounted as per the risk-free discount curve;
· TNE = Target Notional Exposure in credit indices or
single-name CDS;
· F = Shortfall Multiplier, assumed to be constant in this
example;
· lb = Lower Bound cash-out threshold, expressed as a
percentage of the security's notional;
· TL = Target Leverage function.
The aim of the structure is to increase the security's NPV in
order to hit the TRV (a «lock-in» event: in this case, the credit
portfolio is unwound and the proceeds of the transaction are high enough to
cover all future promised coupon, fee and principal payments until maturity by
construction of the TRV aggregate. At the same time, the structure must avoid
any «lock-out» event, which takes place when the security's NPV hits
a fixed percentage lb, usually around 10%, of the security's notional N.
As long as no lock-in nor lock-out event has occured, the
leveraging mechanism described hereafter expresses the Target Leverage function
TL(t) as a linearly increasing function of the structure's shortfall, defined
as the difference between the TRV and the NPV:
NPV (t) = MtM(t) + Collat(t) + CA(t)
TRV (t) = PV Notional(t) + PV Coupons(t) + PV Fees(t) TNE(t) = F
· (TRV (t) - NPV (t))
T NE(t)
T L(t) = A
|
(1.2)
|
|
In other words, the CPDO's leveraging mechanism enables the
structure to increase its credit exposure when the shortfall increases, i.e.
when the security's NPV incurs MtM or default losses: «the more you lose,
the more you bet». Conversely, MtM gains translate into a reduction in the
structure's credit exposure.
Constant Proportion Portfolio Insurance: placing greedy but
secured bets
Originally designed for equity underlyings, Constant
Proportion Portfolio Insurance (CPPI) products referencing credit-linked assets
have developped in the past three years. Unlike CPDOs, CPPIs are
principal-protected at maturity. In other words, the investor will always
receive the notional of the security at its maturity, whereas the CPDO
noteholder can end up with as little as lb% of his initial investment.
The CPPI is a security whose principal is protected at
maturity and whose coupons can be rated by S&P and/or Moody's and/or
Fitch. Similarly to CPDOs, the rated
CPPI pays to the noteholder quarterly EURIBOR/LIBOR coupons
plus a spread around 50-100 bps depending on issuing market conditions. This
return is achieved by selling protection on a portfolio of single-name CDS in
an amount that is adjusted dynamically during the transaction's lifetime. This
dynamic «leverage» function can reach as much as 10-12 times the
initial notional.
Notations introduced earlier to describe the CPDO structure
remain valid hereafter. In addition, we define the following variables at time
t:
· BF = Bond Floor: value of a risk-free zero-coupon bond
maturing at the legal maturity of the security;
· R = Reserve;
· RM = Reserve Multiplier.
A CPPI lock-out event happens whenever the security's NPV hits
the Bond Floor BF. A lock-in event is the same as for CPDOs. The leveraging
mechanism is different however: the CPPI's target leverage function TL is an
increasing function of the Reserve R, defined as the difference between the
security's NPV and BF.
R(t) = NPV (t) - BF(t) TNE(t) = RM · R(t)
TNE(t)
TL(t) = A
|
(1.3)
|
The CPPI's leveraging mechanism enables the structure to
increase its credit exposure when the reserve increases, i.e. when either the
security's NPV increases due to MtM gains or its BF rises as a result of lower
interest rates. The more money you make, the more you can afford losing by
increasing your bets.
Chapter 2
Modelling and pricing CSO
tranches
After choosing the pool of single-name CDS and defining the
characteristics of the CSO tranche (attachment and detachment points), we want
to determine a fair spread to be paid to the tranche buyer (i.e. the protection
seller) as a fair reward for bearing this credit risk so that the present value
of his investment is zero at inception (assuming no transaction costs nor fees
to be paid to the arranging bank). Such a fair spread will eventually depend on
the portfolio's joint loss distribution function accross time horizon L(t)
until the CSO's maturity.
2.1 Modelling a CSO tranche payoff
Given an underlying portoflio of single name CDS, we assume
that we have access to the joint loss distribution function L(t) of the
portfolio at any time t, 0 t T, where T denotes the maturity of the
transaction. We call respectively K and K the attachment and detachment
points of our tranche. Its initial nominal amount is equal to K - K and
the cumulative losses M(t) that affect that tranche at any time t is given by
the following formula:
M(t) = (L(t) - K) - (L(t) - K)
We now assume that the underlying CDS portfolio is made of N
reference obligors, each with a nominal amount An and a recovery
rate Rn for n = 1,2, .., N. Let Ln = (1 - Rn)An be the
loss given default of obligor n. Let rn be the default time of
obligor n. Let Nn(t) = 1{ôn=t} define
the counting process which jumps from 0 to 1 when the nth obligor
defaults. The portfolio loss function L(t) is then given by:
L(t) = XN LnNn(t) (2.1)
n=1
We note that the functions L(t) and therefore M(t) are pure jump
processes.
2.2 Default and premium legs of a CSO tranche
Similarly to the approach presented for valuing the fair spread
of a single-name CDS, we determine the fair premium W * of the CSO tranche
by equalizing the present
value of the default leg DL and the premium leg PL(W) of the
tranche: by definition, W* solves the following equation:
PL(W*) - DL = 0 (2.2)
The existence of a liquid market for standard CSO tranches
based on ITRAXX and CDX indices provides us with a satisfactory framework for
pricing credit default correlation among obligors, hence CSO tranches, under
the risk-neutral probability. From now on, we assume that all expectations are
taken under the risk-neutral probability measure.
2.2.1 Default leg
Given that M(t) is an increasing function, we can define
Stieltjes-Lebesgue integrals with respect to M(t). The discounted payoff
corresponding to potential default payments can therefore be written as:
I0
T n
X ( )
B(0, t)dM(t) := B(0, ôj)Nj(T ) M(ôj) - M(ô-
j )
j=1
Using Stieltjes integration by parts formula and Fubini's
theorem, the price of the default leg under the risk neutral probability
measure can be expressed as:
I T J T
DL = E[ B(0, t)dM(t)] = B(0, T ) E[M(T )] + E[M(t)]dB(0, t)
0 0
2.2.2 Premium leg
Similarly, the price of the premium leg of the CSO tranche
under the risk neutral probability measure is given by the folowing expression,
where discrete premium payments are assumed to take place on
(Tj)j=1..m with T0 is the start date of the tranche and
Tm = T is its legal maturity date.
? ?
m J Tj
P L(W ) = E ? B(0, Tj) W (K - K - M(t))dt?
j=1 Tj-1
Xm j=1
J Tj
B(0, Tj)W (K - K)(Tj - Tj-1) - E[M(t)]dt
Tj-1
2.2.3 Fair premium
We can now solve equation (2.2) for W * as a function of the
expected cumulative tranche loss E[M(t)]:
B(0, T ) E[M(T )] + f 0 T E[M(t)]dB(0, t)
W * = (
>m ) (2.3)
(K - K)(Tj - Tj-1) - f Tj
j=1 B(0, Tj) Tj-1 E[M(t)]dt
As soon as we can compute the expected tranche loss E[M(t)],
the calculation of the tranche fair premium becomes straightforward. In order
to do so, we then have to make further modelling assumptions on the behaviour
of the joint tranche loss distribution M(t), or equivalently L(t).
2.3 The semi-analytic approach: one factor Gaussian
Copula model
2.3.1 Copula functions: basic properties
Copula functions are useful tools for modelling dependency
between random variables, for they allow to separate the univariate margins and
the dependence structure from the multivariate distribution.
Theorem 1. Sklar's Theorem
Let F be a joint distribution function with margins F1, .., Fd.
There exists a copula function C such that for all x1, .., xd in [-8,
+8],
F(x1,..,xd) = C(F1(x1),..,Fd(xd))
Conversely, if C is a copula function and F1, .., Fd are the
margins of respectively X1, .., Xd, then the multivariate function F
of the vector (X1, ..Xd) is such that, for all x1, .., xd in [-8,
+8],
F (x1, .., xd) = C(F1(x1), .., Fd(xd))
If the margins are continuous, then the copula function C is
unique.
We shall need another key result in order to ensure copula
functions are flexible enough to model joint loss distributions:
Proposition 1. Invariance
Let C denote the copula function of continuous random vector
(X1, .., Xd). Let f1, .., fd be strictly increasing functions
defined respectively on the support of X1, .., Xd. Then C is also
the copula function of the continuous random vector (f(X1), .., f(Xd)).
We then recall the cumulative distribution function Ö of
a standard gaussian variable and that of a multivariate standard centered
gaussian vector with correlation matrix R:
x 1
Ö(x) =
f8v2ð
|
e-t2/2dt
|
x1xd11T 0-1y · dy1..dyd
ÖV xd) "
f8- f e2y
Definition 1. Gaussian Copula
Let (X1, .., Xd) be a gaussian vector with correlation matrix R,
zero mean and unit variance. We can then express its copula function
CR as follows:
CR(u1, .., ud) = Ö`V/)
(Ö-1(u1),..,Ö-1(ud))
Given the invariance property of copula functions seen in
proposition (1), CR is also the copula function of any gaussian vector with
correlation matrix R.
2.3.2 The one factor gaussian copula model
Let (ô1, .., ôN) define the random vector of
default times among the N obligors of our reference portfolio. Given
equation (2.1) and under deterministic assumptions for recovery rates,
determining the joint distribution of (ô1, .., ôN) is equivalent
to
determining the joint loss distribution L(t) for all t = T.
We further assume that each default time random variable
ôj, j = 1..N, follows an exponential law of parameter ëj. In other
words, the cumulative distribution function Qj of ôj can be expressed
as:
?t ? [0, T], P(ôj = t) := Q(t) = 1 - e_ëjt
We now wish to model the dependency between those default time
random variables. The current market standard for doing so is to use the
gaussian copula function CR where its correlation matrix R is defined as
follows:
?
? ? ? ? ?
R=
?
?????
1 p ... p
p 1 ..
.. ..
... ...
. .. p
p ... p 1
Applying Sklar's reciprocal theorem, we can then exhibit the
resulting cumulative distribution function Q of the random vector (ô1,
.., ôN):
P(ô1 = t1,..,ôN = tN) := Q(t1,..,tN) = CR
(Q1(t1),..,QN(tN))
A convenient way to simulate the random vector of default
times (ô1, .., ôN) related together by a gaussian copula is to use
an auxiliary random vector (X1, .., XN) modelled upon a single factor approach.
We assume that all Xj, j = 1..N, depend respectively on a common standard
gaussian factor Z and on an idiosyncratic standard gaussian factor Zj, where
all Zj are mutually independent and independent from Z. Conditionnally on the
common factor Z, all Xj, j = 1, .., N are therefore independent.
?j ? [1,..,N],Xj := vpZ + /1 - pZj
Proposition 2. The random vector (X1, .., XN) is a gaussian
vector with correlation matrix equal to R.
Proof.
v/
?
????????
=
...
0 vp
...
...
. ..
0
....
. .. ..
Z Z1
...
·
?
???????
... ZN
?
???????
?
????????
(X1, .., XN) = (vpZ + v/ 1 - pZ1, .., vpZ + 1 - pZN) vp -v1 - p 0
... 0
...
....
. .. .. -v1 - p
0 vp
0 . . .
We have expressed (X1, .., XN) as an affine transformation of
the gaussian vector (Z, Z1, .., ZN). Hence, (X1, .., XN) is a gaussian vector
itself. The general term of its correlation matrix (pij,1 = i, j = N), is given
by:

Cov (vpZ + v1 - pZi,vpZ + v1 - pZj) pij = V ar(Xi)V
ar(Xj)
= äij(1 - p) + p
Hence, the correlation matrix of (X1, .., XN) is also R.
Applying invariance proposition (1) to the random vector of
default times (ô1,.., ôN) Law = (Q-1
1 (Ö(X1)), .., Q-1
N (Ö(XN)), we conclude that both vectors share the same
gaussian copula function.
2.4 Back to CSO tranche pricing: computing the expected
portfolio loss
We shall now present three numerical methods in order to
evaluate the expected tranche loss function E[M(t)], Vt E [0, T]. We stress the
fact that the following methods apply within the framework of the one factor
gaussian copula model to a finite heterogeneous portfolio (i.e. in terms of
individual nominal weights and recovery rates) of obligors with deterministic
recovery rates. We shall not detail the well known analytic results that can be
derived from Large Homogeneous Portfolios (LHP).
2.4.1 Monte-Carlo simulations
The Monte-Carlo approach is probably the most straightforward
method to price a CSO tranche fair premium:
1. Simulate N +1 independent standard gaussian variables (Z,
Z1, ..ZN) by using, for instance, the Box-Müller transform, the polar
method or even by drawing in the random variable Ö-1(U), where
U is a uniform random variable; within the one factor gaussian copula model,
the random vector (X1, .., XN) = ( /ñZ + /1 - ñZ1, .., /ñZ
+ /1 - ñZN) is therefore a gaussian vector with correlation matrix R.
2. Simulate (ô1, .., ôN) by drawing in the random
vector
(Q-1
1 (Ö(X1)), .., Q-1
N (Ö(XN))
3. Evaluate the tranche loss function M(t), Vt E [0, T] along
this loss scenario;
4. Repeat steps 1 & 2 and evaluate the tranche loss function
along this new loss scenario;
5. Loop on step 4 until you feel comfortable (confidence
interval or variance criteria) with the convergence of the empirical estimate
of E(M(t)), Vt E [0, T];
6. Evaluate the CSO tranche fair premium detailed in equation
(2.3). Such a simple anf flexible method comes at a high computation cost
though, because one has to draw millions of random variables in the case of a
reasonably large portfolio (between 100 and 200 obligors).
2.4.2 Evaluating the loss characteristic function
We first determine the expression of pj(t|Z), the cumulative
distribution function of default time ôj, j = 1..N conditional on the
common factor Z.
?j ? {1, .., N}, ?t ? [0, T], pj(t|Z) : = P(ôj = t|Z)
= P (Q6 1(Ö(Xj)) t|Z)
~ = PQ-1(Ö(vñZ + ñZj)) = t|Z)
= P (Zj G 4Ö-1 (Qj(t)) v
ñZ |Z)
(Ö-1(Qj(t)) ? ?ñZ
v1 ? ñ )
- ñ
= Ö
We then derive the total loss characteristic function conditional
on the common factor Z:
?u ? R, ÖL(t)(u|V ) : = E [exp(iuL(t))|Z]
= E [exp(iu ELnNn(t))|Z1
n=1
=
E [fl exp(iuLnNn(t)) | Z1
n=1
YN E [exp(iuLnNn(t))|Z]
n=1
YN [1 + pn(t|Z)(exp(iuLn) -
1)]
n=1
where we have used that (N1(t),..,NN(t)) are mutually
independent conditionally
on the common factor Z.
We now integrate the conditional characteristic function over the
common gaussian factor Z to retrieve the unconditional characteristic function
ÖL(t):
+8
= IÖL(t)(u|z)dÖ(z)
?u ? R, ÖL(t)(u) : = E [ÖL(t)(u|Z)]
Once we have found the loss characteristic function, we can
use the Fast Fourier Transform (FFT) to recover the loss distribution function
itself, which we then plug into equation (2.3) to derive the CSO tranche fair
premium.
2.4.3 Andersen's recursive formula
The recursive approach described in [1] builds on the fact
that the portfolio loss function can only take a limited number of values. This
set of values depends in turn on the obligors' individual loss given default
levels Ln, ?n ? {1, .., N}. We now assume that all those loss levels
can be expressed as multiples of a loss unit l:
?n ? {1, .., N}, ?an ? N, Ln =
anl
?i ? {0,1, ..,
|
XN k=1
|
ák}, P (L = il) = i+8 Pz--z(L(N)
=
L il)dö(z)
|
We further assume that all N obligors are ranked. The possible
values for the loss function L are restricted to the following subset:
{m
L ? E ájkl, m ? {1,..,N}, {j1,..,jm}
? {1,.., N} ? {0} k=1
The power of Andersen's recursive algorithm is that it allows
to compute the loss distribution while assuming that the pool of obligors
results from the sequential addition of all obligors upon one specific ranking
order. Let j ? {1, .., N} refer to the first j obligors added to the pool and
L(j) the discretized loss function associated
with that sub-pool. We can then express the loss distribution of
L(j) as a function of L(j-1).
Proposition 3. Andersen's recursive formula
Let j ? {1, .., N} and L(0) = 0. Assume the loss
distribution function L(j-1) conditional on the common factor Z is known. Let
QZ denote the risk neutral probability measure conditional on the
factor Z and pj the default probability of jth obligor conditional
on factor Z. Then we have the following recursive result:
?i ? {0, 1, .., XN ák},
k=1
QZ (-0) = il) = (1 - pj)QZ(L(j-1) = il) +
pjQZ(L(j-1) = (i - áj)l)
Proof. Let Dj,j?{1,..N} denote the default indicator variable
of jth obligor conditional on the factor Z. Using the conditional
independence of Dj,j?{1,..N}, we can then write for all j ? {1, ..,N} and for
all i ? {0, .., Ejk=1 ák}:
QZ(L(j) = il) =
QZ(L(j-1) = (i - áj)l,Dj = 1) + QZ(Lj-1
= il, Dj = 0)
= QZ(L(j-1) = (i - áj)l)QZ(Dj = 1)
+ QZ(L(j-1) = il)QZ(Dj = 0)
= pjQZ(L(j-1) = (i - áj)l) + (1 - pj)QZ(L(j-1) = il)

Andersen's recursive formula evaluated at rank N thus provides
the conditional loss distribution L(N). The last step in the
computation of the unconditional discretized loss distribution L is to
integrate the conditional loss distribtion against the density function of the
factor's standard gaussian law:
Chapter 3
Modelling and Rating Dynamic
Proportion Portfolio Insurance
products
Summer 2007's turmoil in global credit markets resulted in a
significant increase in volatility, thereby threatening the rating stability of
many existing structured products including CSO tranches and CPDOs. A
straightforward response to such a volatile environment is to cap the downside
Mark-to-Market risk by adding a capital protection feature to new structured
products: Constant Porportion Portfolio Insurance (CPPI) products and their
most recent offshoots, Dynamic Proportion Indurance Products (DPPI), belong to
that category.
We shall first recall the main principles of Moody's approach
for measuring risks in order to rate CPDO and CPPI/DPPI products and outline
its main assumptions in modelling risk factors. We shall then describe the
DPPI's major risk sensitivities and present some of its key structuring
features in order to mitigate those risks. Finally, we shall analyze the DPPI's
behaviour under several stress-scenarios.
3.1 Moody's approach to rating CPDO and CPPI/DPPI
products
3.1.1 Historical vs risk-neutral probability measures
Before going into the details of rating and pricing DPPI
products, we shall address the following question: why do investment banks
price their structured products under a risk neutral probability measure while
rating agencies rate them under the historical probability measure?
Rating agencies evaluate loss ditributions under the
historical probability measure because investors are mainly concerned with
knowing how likely it is that they are going to lose money in our real
«historical» world. They don't care about such a likelihood in a
risk-neutral world. Doing so requires rating agencies to estimate future
historical default probabilities and loss distributions, the parametres of
which are calibrated statistically, whenever it is possible, on past historical
data.
On the other hand, investment banks are concerned with pricing
such products by evaluating the associated hedging costs. Fundamental results
such as HarrissonPliska's no-arbitrage pricing theorem and Black-Scholes
conclusions ensure that:
· in a viable and complete market, there exists only one
probability measure Q called «risk-neutral» under which discounted
asset prices are martingales;
· there exists a self-financing portfolio that replicates
the product's payoff.
Girsanov's theorem allows us to relate historical and risk
neutral probability measures through the notion of risk premium, which in turn
can be interpreted in terms of risk aversion: under most market circumstances,
real-world investors are naturally risk-averse and hence require to be paid an
extra return for bearing default risk as compared to its true historical
insurance cost. Hence coexisting historical and risk-neutral probability
measures serve different purposes: the historical approach prevails for
weighting future real-world scenarios and building risk measures such as the
Value-at-Risk, while the risk-neutral framework allows the pricing and the
hedging of traded securities.
3.1.2 Moody's Metric and coherent risk measures
We now temporarily put the risk-neutral measure aside and
focus on the historical probability measure. Moody's uses the same methodology
to rate CPDO and CPPI/DPPI products. It estimates the expected present value of
the loss function L(M) through Monte-Carlo simulations under the historical
probability measure.
Definition 2. Moody's expected discounted loss
Let t E I = {0, ät, .., kät, .., T} describe the
discrete time scale with T the maturity of the deal. Let Xt :=
(X(1)
t , .., X(p)
t ) be the p-vector of risk factors observed as of date t. We
introduce the filtration (Ft){t?I} defined as ?t E I, Ft := ó
(Xu,u E I,u = s). We further define the three stopping times (r, r,
r):
r := inf{s = 0, NPV (s) = TRV (s)}
r := inf{s = 0, NPV (s) = BF(s)} A T r := r A r
We then express Moody's risky discount factor DF(M) as
a function of the EURIBOR/LIBOR rate curve and of the senior spread s served to
the investor:
?t E I, DF(M)(t) :=
|
t-1Y i=0
|
1
|
1 + ät(EUR(iät, (i + 1)ät) + s)
|
>1p i=1 L(M) L(M) = i
p
|
+
|
ót99%
|
vp
|
Then, under the historical probability measure, Moody's expected
discounted loss L(M) is given below:
[ ]
L(M) := E 1{ô<ô} [A(1 - sl) - max(NP V
(r), BF (r)) + DI(r)]+ DF (M)(r)
where sl is the detachment point in % of the subordinated
note.
In practise, Moody's uses an unbiased empirical estimator of
L(M), L(M) defined as:
where t99% := Ö-1(99%), p is the number of
Monte-Carlo simulations, L(M)
i is the
loss calculatd on the ith scenario and
ó is the standard error of (L(M)
1 , .., L(M)
p ).
Moody's then maps that value L(M) and the maturity of the deal T
against a positive real scale S = [0, 21] through a function MM called
«Moody's Metric»:
MM : [0, 1] × R+ -? [0, 21]
(x,t) i-? MM(x,t)
We shall now describe how the Moody's Metric mapping function
works. We first define the letter-to-integer mapping function R:
R : {Aaa,Aa1,..,Ca,C} -? {1,..,21}
m -? R(m)
The discrete mapping table is given below:
Rating-Figure
|
Rating-Letter
|
1
|
Aaa
|
2
|
Aa1
|
3
|
Aa2
|
4
|
Aa3
|
5
|
A1
|
6
|
A2
|
7
|
A3
|
8
|
Baa1
|
9
|
Baa2
|
10
|
Baa3
|
11
|
Ba1
|
12
|
Ba2
|
13
|
Ba3
|
14
|
B1
|
15
|
B2
|
16
|
B3
|
17
|
Caa1
|
18
|
Caa2
|
19
|
Caa3
|
20
|
Ca
|
21
|
C
|
Figure 3.1: Moody's rating conversion table
We shall now define the discrete function EL that maps the
integer equivalent of a rating category and a maturity with a percentage
expected loss:
EL : {1, .., 21} × {1, .., T} -? [0, 1]
(m, t) '-? EL(m, t)
Moody's calibrates the function EL on historical default data by
using the cohort method.
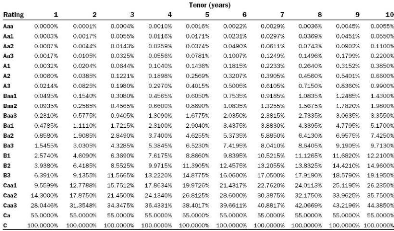
Figure 3.2: Moody's idealized EL values by rating category and
tenor
We shall then define EL, the time-continuous version
of EL function obtained by linearly interpolating EL between two discrete
integer dates:
EL : {1,..,21} x [0,T] -*[0,1]
(m, t) i-* (t + 1 - [t])EL(m, [t]) + (t - [t])EL(m, [t] + 1)
Let us now define the reverse mapping function F -1
that transforms any percentage loss level and tenor into a rating:
F -1 : [0, 1] x [0, T] -* {1, .., 21}
(x,t) -* min{m E {1,..,21}| EL(m,t) = x}
We finally give the expression of the Moody's Metric function MM:
Definition 3. Moody's Metric
?x E [0,1],?t E [0,T],
EL(F -1(x, t), t))
ln x - ln (
MM(x, t) := F -1(x, t) + ln ( EL(F
-1(x, t) + 1, t)) - ln ( EL(F-1(x, t), t))
In other words, the Moody's Metric can be seen as a
standardized continuous scale that allows to compare expected loss levels for
different tenors. We shall now take a closer look at the notion of risk measure
and understand to what extent it makes sense to use the expected loss as a
proxy for measuring risk.
Definition 4. Coherent Risk Measure
Let C denote a set of random variables representing all
possible risky positions and L E C be a random variable whose range of values
represents possible losses from any given risky position. We define the risk
measure function p as a mapping from C to R. The risk measure p is coherent if
it is:
i) monotonous: ?X, Y ? G, X = Y p(X) = p(Y )
ii) positively homogeneous: ?X ? G, ?h > 0, hX ? G and p(hX)
= hp(X)
iii) sub-additive: ?X, Y ? G, X + Y ? G and p(X + Y ) = p(X) +
p(Y )
iv) translation invariant: ?X ? G, ?a ? R s.t. X + a ? G, p(X +
a) = p(X) + a
Proposition 4.
If G+ is a set of non-negative random variables,
interpreted as a loss from a risky position, then expected value is a coherent
risk measure:
?X ? G+, p(X) := E[X]

Proof. Properties (i),(ii), (iii) and (iv) immediately result
from the expectation's linearity.
Unlike E[X], the Moody's Metric MM(X, t), where X is a
positive random variable that takes its values in [0, 1], is not a coherent
risk measure: though it is clearly monotonous and subadditive (because MM(., t)
is increasing and concave), it is neither positively homogeneous, nor
translation invariant.
3.2 Modelling risk factors
Moody's Monte-Carlo approach requires risk factors to be
modelled and simulated. The complexity of the task for rating DPPI products
comes from the fact that risk factors are numerous and can depend on each
other. We assume that the DPPI's portfolio is initially composed of long CDS
positions on N obligors. The 5Y CDS mid-spread and the rating of each obligor
n, n ? {1, .., N}, are known at the deal's inception and are equal to
(sn(0),Rn(0)).
3.2.1 Credit spread processes influenced by defaults and
ratings
Moody's assumes that individual 5Y CDS spread processes follow
a generalized Vasicek diffusion process specific to rating groups. The 21
available rating categories are grouped into 8 rating groups {Aaa, Aa, A, Baa,
Ba, B, Caa, Ca/C}. Let j ? {1, .., 8} denote the rating group's index. Then
(S(t) = (S1(t), .., S8(t)))t?[0,T] is the associated 8-dimensional spread
random process. We then give the stochastic differential
equation ruling the spread process (S(t)), where (á,
â, ã = 1, ó, ó, ADR, ADR, a, b, p) are
historically calibrated parametres of the diffusion process and t0 is equal to
1 year:
?j ? {1, .., 8}, ?t ? [0, T], dSj(t) =
á(â - Sj(t))dt + min(ó,
óSãj (t))dW (j)
t
where
|
? ?????
?????
|
â = â min (ADR,max (ADR, aADR(t) + b)) if t =
t0
PN n=1 Ln(Nn(t)--Nn(t--t0))
ADR(t) = PN if t = t0
n=1 An
â = â if t < t0
?(i,j) ? {1, .., 8}2, d (W(i),
W(j)) t = pijdt
|
(3.1)
|
and Sj(0) = Sj,0
Equation (3.1) is remarkable for several reasons. First, given
that ã = 1 and that the spread process is continuous, it doesn't
allow credit spreads to be negative. Second, it models a dependency between
the spread process itself and default events through
the parametres (ADR, ADR) and the variable ADR(t): the
idea is to make the current long term mean 3 depend on the portfolio's Average
Default Rate ADR(t) such that 3 is stressed for a limited time period equal to
t0 after any event of default and tightens in default-free environments. Third,
it accounts for a dependency between the obligor's rating and its spread
process: whenever an obligor's rating group changes, its associated spread
process is updated accordingly. Fourth, the random noise source (Wt = (W1(t),
.., W8(t))) is a correlated 8-dimensional brownian motion.
Such dependency relationships are far from being flawless
though: one could argue that they do not account for default events occuring
outside the portfolio's pool of obligors. One could also demonstrate that
individual spread processes are not driven by their belonging to a rating
group, but more by marketwide, firm or industry specific events that are not
necessarily reflected in a rating change.
In order to simulate a CDS spread for any tenor, Moody's
assumes that the term structure of spreads is deterministic, calibrated on
historical data and specific to each one of the 8 rating groups defined above.
Though such an assumption may seem highly questionable at first and lead to
obvious arbitrage opportunities, Moody's solves the issue by requiring stress
scenarios to be run with a flat term structure while preserving a stressed
Moody's Metric level.
3.2.2 Rating migrations and default events
Moody's simulates rating migrations and default events within
a multi-factor gaussian copula framework applied to a markovian multi-period
rating transition model. The first input of this model is a square rating
transition matrix over a given time horizon T, noted MT E Mp(R),
where p denotes the number of potential rating categories of the obligors,
including one default category. Moody's assumes there are 18 of them, the
mapping of which can be derived from figure (3.1) with categories Caa - C
merged and an extra default category D with rating 18.
The rating path of the nth obligor, j E {1, .., N},
until time horizon T is given by the random process (Rn(t))t?[0,T]:
Rn : Ù × [0,T] -? {1,..,18} (ù,t)
-? Rn(t)(w)
We then recall the definitions of a generator matrix and of a
time-homogeneous Markov process
Definition 5. Generator Matrix
Assuming A E Mp(R) with general term
(ëij)(i,j)?{1,..,p}2. Then A is called a generator matrix
if:
i) Vi E {1, ..,p}, >ip j=1 ëij = 0
ii) V(i,j) E {1,..,p}2, i =6 j ëij = 0
Definition 6. Time-homogeneous Markov process
X is a time-homogeneous Markov process with generator Ë
if:
?t = 0, ?Ät > 0, ?(i, j) ? {1, .., p}2,
P(X(t + Ät) = j | X(t) = i) = (eËÄt)ij
We now assume that (Rn(t))tE[0,T] is a Markov
time-homogeneous process with generator matrix Ë. We introduce the
transition matrix MÄt over time period Ät through its general term
(pij)(i,j)<p:
?t ? [0, T, ]?(i, j) ? {1, .., p}2, pij := P
(Rn(t + Ät) = j | Rn(t) = i)
It is worth noting that pij does not depend on t because of
the time-homogeneous property of (Rn(t))tE[0,T]. As a direct
consequence of Rn's definition, we have the following property:
Proposition 5. Composition of transition matrices
Assume Ät is such that TÄt ? N*.
Then:
T } k = MTTÄt
?k ? {1, ..
Ät
We shall now describe briefly Moody's multi-factor gaussian
copula model: similarly to the one factor gaussian copula model, the idea is to
draw a random vector X = (X1, .., XN) from a gaussian law with a given
correlation matrix Ó, where the latter depends on several factors. Let
us define (ZG, ZI, ZI,R) as three independent
standard gaussian factors that account for respectively the global state of the
economy, the state of any specific industrial sector and for a combination of
both industrial and regional factors. For any given obligor n ? {1, .., N}, let
us define en as an idiosyncratic factor that follows a standard
gaussian law and that is independent from the common factors (ZG,
ZI, ZI,R) and from all other idiosyncratic factors. Then,
for all n ? {1, .., N}, one can affect the state variable Xn to
nth obligor:
qXn ñG ZG
ñInZI VñIn ,R ZI,R \
+ 1 - ñG -ñIn -ñn I,Ren
The random vector X is a gaussian vector with zero mean and a
correlation matrix Ó given below. The correlation parametres
(ñGn , ñIn, ñn
I,R) are specific to each obligor and depend on some characteristics
of their businesses in terms of industry and operations' scale. They are picked
up from a subset of values subject to Ó remaining positive definite.
?
? ? ? ? ?
Ó=
?
? ? ? ? ?
1 ñ12 . . . ñ1p
.
.
ñ21 1 .
. .
.
...
...
. ..ñp-1,p
ñp1 . . . ñp,p-1 1
with:
q? (i, i) ? { 1, .., p}2 ñij ñG
Vñi ,Rñj,R
Applying well known results on the generalized invert of the
distribution function of (Rn(t + Ät)|Rn(t)), we can
write the following proposition:
Proposition 6. Rating transition simulation
Let us assume that each obligor's rating is likely to be
confirmed or revised only on
the following dates {Ät,..,kÄt,..,mÄt}, where m :=
T/Ät ? N*. Let F -1
k,Ät, k ?
{1, ..,p} denote the generalized invert of the cumulative
distribution function of rating transitions over time period Ät starting
from initial rating category k. We recall that Ö is the
cumulative distribution function of the standard gaussian law and that
(X1,..,XN) is the gaussian vector with correlation matrix Ó
describing the state of our N obligors. We finally assume that initial
ratings (R1(0),..,RN(0)) are known. Then the rating of each obligor on
discrete dates {Ät,..,kÄt,..,mÄt} can be expressed through the
recursive formula:
?k ? {1, .., m}, ?n ? {1, .., N}, Rn(kÄt)
= FR,1:((k-1)
Ät),Ät (Ö(Xn))
Moody's uses its historical database of rating transitions and
defaults over time horizon T to build the marginal cumulative distribution
functions (Fk,T)k?{1,..,p} and MT through some cohort method. Assuming
(Rn(t))t?[0,T] is a Markov time-homogeneous process, one can infer
MÄt thanks to proposition (5) and use proposition (6) to simulate N
correlated rating paths. The rescaling of matrix MT comes at a cost however:
given the choice of the gaussian copula, one can show that when Ät --? 0,
joint default times (ô(Ät)
1 , ..,ô(Ät) N) become independent: a
way to address this issue is to stress Ó as Ät gets smaller so that
the correlation structure is somehow preserved. In the case of the DPPI, T is
equal to 10 years and Ät to 6 months.
3.2.3 Interest rates process and other parametres Interest
rates
Moody's interest rate model is based on projecting a daily
evolution of 3-month and 10-year term rates and linearly interpolating between
them for rates of other tenors. Rates with tenors shorter than 3 months are
assumed to be equal to the 3-month rate. 3-month and 10-year term rates follow
a two-dimensional correlated Cox-Ingersoll-Ross (CIR) process, where
Rs and Rl denote respectively 3-month and 10-year term
rate processes:
?
??? ?
????
dRst = ás(âs --
Rst)dt + ópRs tdW s
dRlt = ál(âl --
Rlt)dt+ó
JRltdWtl
d (Ws,Wl)t = ñdt
(Rs0, Rl 0) = (rs, rl)
In order to make sure that Euler's discretized sheme does not
generate negative values for interest rates, the natural discretized CIR
process is given below:
(Rs0, Rl0) = (rs,
rl) ?k ? {1,..,T/Ät},
?
?? ?
???
(3.2)
Rs(kÄt) = |Rs((k -- 1)Ät) +
ás(âs -- Rs((k -- 1)Ät))Ät + ..
+ ÄtRs((k -- 1)Ät)Z1|
Rl(kÄt) = |Rl((k -- 1)Ät) +
ál(âl -- Rl((k -- 1)Ät))Ät + .. +
ó0/ÄtRl ((k -- 1)Ät)(ñZ1 +
ñ2Z2)|
Recovery Rates
Default recovery rates for our N obligors are assumed to be
random and follow marginal Beta distributions correlated through a one factor
gaussian copula model. Given that the recovery rate RRn of each
obligor n follows a Beta distribution, it is characterized by its mean
lin and standard deviation ón. lin and
ón depend on the obligor's location, its type (corporate,
sovereign,..) and the seniority of the CDS underlying reference obligation. The
parameters án and On of the
Beta(án, On) distribution are given
below:
2 1-u
?n ? {1, .., N}, { án = lin
ó2 1411-un 1 \
)
On = (1 -- lin)(lin ón 2 1
Let RRG Law = N(0,1) denote the global recovery factor. The
standard normal variable Xn describing the recovery rate of obligor
n is given by:
Xn = V pGRRG + V1 -- pGen
where pG is a correlation parametre common to all
obligors and en Law = N(0,1) the idiosyncratic recovery factor independent from
the common factor RRG and from all other idiosyncratic ones. The
following proposition allows us to simulate a N-vector of recovery rates drawn
from marginal Beta distributions correlated through a one factor gaussian
copula:
Proposition 7. Recovery Rates Simulation
Let F;771 denote the invert of the
cumulative distribution function of Beta(án,
On)
law. Assume (RRG, e1, .., €N) Law = N (0,
IN+1). Then the distributions of individual recovery rates are
given by:
?n ? {1, .., N}, RRn Law= Fn 1 (Ö(V pG
RRG + V1 -- pGen))
3.3 Portfolio investment rules
We shall now present the major characteristics of the DPPI
that allow us to significantly improve the rating of the basic CPPI. The DPPI
indeed capitalizes on several structural features and investment rules in order
to achieve the target rating of Aa3 over a time horizon of 10 years.
3.3.1 Dynamic leverage function
The Target Notional Exposure at time t, noted TNE(t), is no
longer a constant multiple of the Reserve at time t, R(t), but a more
complicated function designed to take advantage of various market conditions.
For doing so, we need to define intermediary variables.
Duration of a CDS contract in a simplified intensity model
The duration D of a CDS contract of constant market spread s
and tenor T years with coupons being paid every Ät year, with
ÄtT := p ? N is given by the following formula:
p E
i=1
D=
ÄtB(0, iÄt)S0(iÄt)
where we assume that the survival function S0(t) := 1 -
P(ô = t) is continuous, differentiable and solves the following
ordinary differential equation with ë(t) ? R+*:
~ S0 0(t) + ë(t)S0(t) = 0
?t ? R+*,
S0(0) = 1
As a result, S0(t) = e-f0 t ë(s)ds. A way to empirically
determine the function ë is to assume that ë is piecewise constant
between all liquid tenors and to use the CDS valuation equation (1.1) to
determine ë recursively: the first step will be to determine ë(T1)
where T1 is the shortest tenor, whith ë(T1) = s(T 1)
1-R after simplifying equation (1.1) with T = T1. The second
step is to extend the maturity of equation (1.1) from T1 to T2, and express
S0(T1) and S0(T2) as a function of respectively ë(T1) and ë(T2). From
that equation, we infer ë(T2), and so on and so forth. R can then be taken
equal to 40% as as market convention and the spread s(Ti) can be read from
market quotations.
Target Notional Exposure function
We easily generalize the initial duration D of a CDS at time t
= 0 with tenor T to the duration function D(t) at time t = T and define the
average duration function D(t) of all long CDS positions in portfolio at time t
by simply taking the weighted arithmetic average of the durations of all single
CDS in portfolio. We further introduce the weighted average rating-dependent
risk function SR(t), which is homogeneous to a CDS spread:
PN n=1 An(t)C(Rn(t))
?t ? [0,T], SR(t) :=
PN n=1 An(t)
where C is an increasing mapping function from integer rating
categories {1, .., 18} to [0, 1] and where An(t) denotes the weight
of obligor n at time t expressed in units of initial notional A (>N n=1
An(t) = A).
We can then describe our dynamic target multiplier TM(t)
function:
1
?t ? [0, T ], T M(t) :=
DR + SR(t)D(t)
where DR is a risk parametre accounting for a portfolio average
default risk.
We then define the average 5-year market spread of the
portfolio at time t, S(t), by simply computing the weighted average 5-year
market spread of all obligors. We then define the piecewise constant
opportunity leverage mapping function OL(t) that maps the weighted average
5-year market spread S(t) with the leverage factor OL(S(t)).
We further introduce two path-dependent multiplying functions,
bu(t) and bd(t), that act respectively as exposure boost-up and
boost-down features:
I bu if t = tu and
max{v?[t-tu,t]}(S(v)) - min{v?[t-tu,t]}(S(v)) =
Äsu bu(t) = 1 if not
{ bd if t = td and S(t) - S(t - td) = Äsd
bd(t) = 1 if not
where (bu, tu, Asu,bd,td,
Asd) are ad-hoc structural parametres. The rationale for introducing
bu(t) and bd(t) is to make the structure proactive in both low and
high volatility spread environments.
Hence we can define the Target Notional Exposure function:
Definition 7. Target Notional Exposure
With notations introduced earlier, the Target Notional Exposure
function behaves according to the following formula:
?t ? [0, T], TNE(t) := bu(t)bd(t) min {OL(t)A, T
M(t)R(t)} Notional Exposure function
As a result, the exposure of the CDS portfolio is either
increased or decreased depending on whether the current Notional Exposure NE(t)
is far enough from the Target Notional Exposure TNE(t):
Definition 8. Notional Exposure
With notations introduced earlier, the Notional Exposure function
behaves according to the following formula:
?t ? [0, T],
NE(t+) = NE(t-)1{ TNE(t)
+ TNE(t)1{ TNE(t)
NE(t
?[(1-lb),(1+lu)]}

NE(t-) /?[(1-lb),(1+lu)]}
where lu ? [0, 1] and ld ? [0,1] are upper and lower
leverage readjustment bounds. 3.3.2 Deferred coupons
One of the core features of the DPPI is its ability to defer
interest payments to investors when its NPV is deemed not to be high enough.
The following recursive formula gives the potential interest payment to be
made on any coupon payment
date kAt, with TÄt ? N* and k ? {1, ..,
T Ät}.
Definition 9. Deferred Interests
Assume t ? {0, At, 2At,..,T}. Let EUR(t,t + At) denote the
EURIBOR rate observed in t with tenor At. Let us introduce the flow
variable:
x(t) := [R(t) - NE(t)u(t)]+
where u(t) is a real parametre ? [0,1]. We then define:
(IP(t), DI(t-),
DI(t+)){t?{0,Ät,2Ät,..,T}
as being respectively the interest payment on date t, the
deferred interest balance just before t and right after t. The
following recursive formula relates all three variables:
(IP (0), DI(0-), DI(0+)) = (0,0,0)
?t ? {At, 2At, .., T},

IP(t) = min {DI(t-) + AAtEUR(t - At, t), x(t)}
DI(t+) = DI(t-) + AAtEUR(t - At, t) -
1{IP(t)=0}IP(t) DI(t-) = DI(t+ - At)(1 +
AtEUR(t - At, t))
3.3.3 Other key structural features
CDS tenor choice
Another crucial structural feature of the DPPI is the tenor
investment rule that allows to adapt CDS tenors depending on market spread
levels. Let us call e(S(t), t) the potential tenor of any CDS investment done
on date t, where e is defined below:
e : [0, 1] x [0, T] -? {3, 5, 7, 10} (x,t) 7-? e(x,t) Removal
of downgraded assets
In order to manage the default risk inherent in owning
leveraged long CDS positions, we shall apply a specific asset-removal rule
based on rating observations: as soon as an obligor's rating has been staying
below a given threshold, say Ba2, for more than 3 straight months, then all CDS
long positions on that obligor must be removed from the portfolio and replaced
by equivalent positions on another obligor whose rating is investment grade,
i.e above or equal to Baa3.
Early cash-in events
The DPPI structure shares with earlier CPPI products an early
cash-in feature that allows the deal to be unwound before the scheduled
maturity date if the deal's NPV is high enough to cover all future liabilities,
i.e future coupons, fees, and principal payments, until the scheduled maturity
date (10 years). However, our DPPI incorporates two extra early cash-in
triggers based on shorter maturity dates, namely 5 years and 7 years. Those
three barrier conditions allow the structure to avoid adverse scenarios where
the NPV would plummet and break the bond floor, hence cash-out, after reaching
its TRV level.
Subordinated note
We add to the DPPI structure an sl := 2% thick subordinated
note, the payoff of which is similar to that of a CSO equity tranche. The
relatively high yield served on that tranche, 250 bps, compensates the
subordinated noteholder for bearing the first loss risk of the structure.
3.4 A study of the DPPI's sensitivities
Given Moody's modelling and calibrating assumptions on risk
factors, we wish to study the DPPI's behaviour as a function of its main
structural features, such as the spread over EURIBOR served to the senior
investor, the CDS tenor investment rule or the parametres of the Target
Notional Exposure function.
Unless otherwise stated, we base our analysis on the portfolio
described in figure (12), on the DPPI optimized parametres listed in figure
(11) on the set of Moody's parametres listed in figures (8), (9) and (10). All
numerical results are based on C++ code developped by both Bear Stearns and
Moody's. As a starting point we plot hereafter the DPPI's simulated discounted
loss and lock-in times distributions based on 30,000 draws..
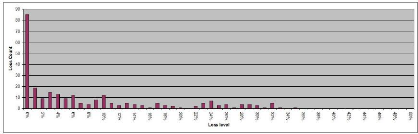
Figure 3.3: DPPI base-case loss distribution conditional on the
structure not cashing in
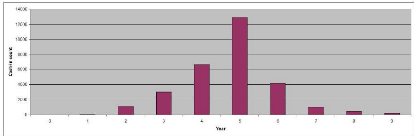
Figure 3.4: Distribution of cash-in times
The Moody's Metric of the base case scenario is 2.697, which is
equivalent to an Aa2 rating.
3.4.1 Tailor-made structural features to achieve target
rating
Senior rated coupon level
The level of the senior rated coupon is obviously a key
parametre in achieving the target rating. The graph below plots the estimated
expected loss and Moodys Metric as a function of S, the senior rated premium
paid above EURIBOR rate and measured in basis points. One shall point out the
jumps in the graph, stemming from the various triggers introduced in the
pay-off loss function L. The rather linear relationship outside jumps is also
fairly straightforward: between two jumps, the subset of cash-out scenarios is
fixed. However, given that the bulk of the loss is explained by scenarios that
cash-out at maturity without paying any single coupon, one would find that the
average loss on those scenarios is proportional to the senior coupon level, as
is the price of a bond paying EURIBOR plus the senior coupon.
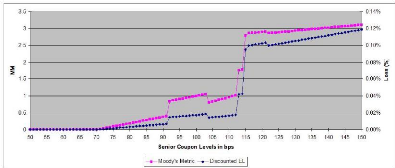
Figure 3.5: Estimated expected loss as a function of S. CDS tenor
choices
Initial and reinvestment CDS tenors have a significant impact
on the shape of the loss distribution and eventually on its expected level. We
now assume that e(.,.) is constant and equal to 0. We then plot the expected
loss level as a function of 0 E {3, 4, .., 10}. The reverse-bell shape of the
diagram accounts for the fact that:
· for short tenors, the lower MtM volatility of the DPPI
does not fully compensate for the loss in contracted CDS spread premia due to
the upward sloping shape of the term structure;
· for long tenors, the gain in contracted CDS spread premia
does not fully offset the impact of a higher MtM volatility.
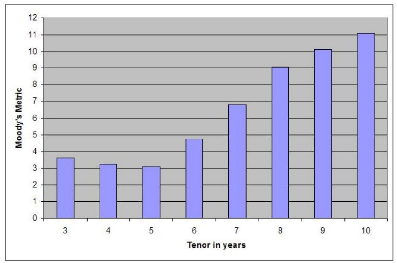
Figure 3.6: Estimated expected loss as a function of è
(2000 simulations per coupon level)
Target Notional Exposure parametrization
In order to get the intuition on how the expected loss behaves
with respect to the Target Notional Exposure function TNE(.), one shall
slightly simplify the latter and assume that opportunity leverage and target
multiplier functions, OL(.) and TM(.),
are constant and equal to respectively OL and TM. We then plot
TNE as a function
of both parametres OL and TM. S1 stands for TM = 20 and S9 for
TM = 40 with Si+1 - Si constant.
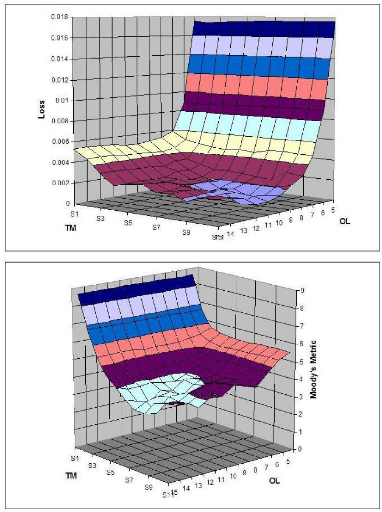
Figure 3.7: Loss and Moody's Metric as a function of OL and TM,
1000 simulations per couple of parametres
One shall point out from the graphs that even with the best
combination of fixed
Opportunity Leverage OL and Target Multiplier TM parametres,
the structure's Moody's Metric remains significantly higher than 2.697
obtained with dynamic OL
and TM functions. Also, we clearly see that there is a
tradeoff between OL and TM that allows the structure to improve its rating:
too high values for both parametres lead to a sub-optimal leverage function,
reflecting the fact the extra MtM volatility
induced by a higher leverage has a negative fat tail effect on
the loss distribution L(M).
3.4.2 Hypothetical stress-scenarios
Being aware of some obvious limitations in its modelling of
risk factors, Moody's requires the DPPI to achieve the target rating in the
base-case scenrio and to pass looser target Moody's Metrics in a series of
stress-scenarios. Major ones are analyzed hereafter: results are shown in table
(3.1).
Credit crisis: systemic brutal spread widening
In order to assess the impact of a brutal widening in CDS
spreads, Moody's requires the market spreads of initial long CDS positions to
be bumped by á = 25% immediately after the deal's inception.
Equivalently, this adverse MtM impact ÄMtM(0) can be expressed in terms of
an upfront haircut to be subtracted from the deal's initial notional A:
ÄMtM(0) = á
|
XN n=1
|
sn(0)An(0)
A TNE(0) · Dn(0)
|
|
where 0 denotes the initial CDS tenor and Dn(0)
the initial duration of the nth CDS. Given initial market conditions
and structural features provided in figures (12) and (11), we compute
ÄMtM(0) = 3.83% and add this haircut to the initial upfront fee of 1%
charged by the arranging investment bank. Results are shown in table (3.1)
Bullish but punctually volatile credit markets: low spreads
stressed by punctual default events
The rationale for testing the DPPI in such mixed market
environments is to test whether the structure can sustain below-average market
spreads, meaning that it does not receive enough CDS premia to cover its
liabilities towards investors (the structure is then deemed to be in
«negative carry»). To do so, Moody's acts on the parametres of the
credit spread process detailed in equation (3.1): it lowers arbitrarily the
long term mean â by decreasing ADR as well as b, and divides the
volatility parametres ó and ó by two. Results are shown in table
(3.1).
Short term default risk concerns: flat credit spread term
structure
As seen earlier, the increasing term structure of credit
spreads is assumed to be deterministic and to result from a shaping function
specific to each rating group. Such an upward slope translates into a positive
time-decay when holding long CDS positions. This increasing term structure gets
attenuated as ratings get worse: it reflects the fact that conditional on the
obligor not defaulting in a near future, its survival probability in the long
run is not worse than presently. Moody's cancels that overall positive
time-decay effect by assuming a flat credit spread term structure guided by
5-year CDS spread levels. Results are shown in table (3.1).
?n E {1,..,N}, ?t E [0,T], ?0 E [0,10], sn(t,t + 0)
= sn(t,t + 5)
Hypothetical stress-scenario results
Scenario
|
L(M)
|
Moody's Metric
|
Rating
|
Base case
|
0.089%
|
2.697
|
Aa2
|
Early credit crisis
|
0.248%
|
4.219
|
A1
|
Low spreads
|
0.447%
|
5.276
|
A2
|
Flat term structure
|
0.685%
|
6.091
|
A3
|
|
Table 3.1: DPPI behaviour under base-case and stress scenarios,
20,000 simulations each except for base-case 30,000 draws
As expected, the DPPI's rating worsens in stressed scenarios.
The structure's resiliency to adverse market environments is obvious: the
magnitude of the potential rating to downgrade is limited to 4 notches in the
flat term structure scenario.
3.5 DPPI: any hidden pricing issue?
So far, we have not raised any pricing nor hedging issue
pertaining to DPPI products. We shall briefly address both points hereafter.
3.5.1 From the investor's perspective
Initially, the return served to the investor can be split
into two separate pieces: the LIBOR/EURIBOR component results from the
structure being long a risk-free bond paying an interbank 3-month rate, while
the extra risky spread of 100 bps is generated by a long leveraged CDS
portfolio. Later on though, the structure may have to realize MtM losses due to
a deleveraging signal sent by the portfolio investment rules: in that case, the
posted collateral must be partly sold in order to pay for the potential loss on
the unwound CDS positions. As a result, the structure is exposed to two major
market risks:
· one is the credit risk inherent in holding long CDS
positions;
· the other is the interest rate risk created by the
potential selling of the collateral before its due term; similarly to what is
being done for CSO structures, such a risk is hedged by entering into 3-month
forward rate agreements rolled every quarter.
As a result, the DPPI's NPV boils down to being equal to the
sum of the par-value of the posted collateral and of the MtM of the long CDS
positions. Moreover, from an investor's perspective, it is sensitive only to
underlying CDS spread levels and not to interest rates, although the
structure's rating will depend on the interest rate curve through its sizing
effect on the Reserve R(t).
3.5.2 From the investment bank's perspective
Unlike CSO tranches, which require delta-hedges to be adapted
dynamically, DPPI products do not need any hedging as such. The only market
risk that is not passed to the investor and that is kept within the bank's
book is the so-called «gap» risk: in case
of a cash-out event, the bank guarantees the bond floor value
to the investor while the structure's NPV may have jumped well below that value
due to overnight volatile market conditions. In other words, pricing that
initial risk GR(0) (for notation convenience) requires to evaluate quantities
such as:
GR(0) = E [(BF(ô) - NPV
(ô))1{ô<ô, NPV
(ô)<BF(ô)}B(0,ô)] (3.3)
Given that default processes are pure jump processes, NPV (.)
is not continuous either: consequently, GR(0) is not equal to 0. Writing a
payoff function such as in equation (3.3) is easy but remains theoretical
though: in practise, one would have to assess dependency relationships between
interest rate risk factors, which mainly impact the structure's bond floor
BF(t), and credit spread levels, which drive the structure's NPV. In other
words, pricing each payoff component of the DPPI is a challenging task that can
only be addressed in simplified frameworks such as the one described in [25]
(time-continuous processes with discrete trading dates).
Another way of evaluating that gap risk is to look at the
potential MtM impact of names defaulting in the portfolio when the NPV gets
close to the Bond Floor BF: by construction, the function TM(t)R(t) has a
mitigating impact on that risk: as the Reserve R(t) shrinks, TM(t)R(t) starts
driving down the Target Notional Exposure TNE(t), hence reducing the MtM impact
of any potential default. More generally, assuming all N names in portfolio are
equally weighted with identical deterministic recovery rates RR and that
readjustment bounds lu and ld are set to 0, we can express the MtM
impact of one default ÄMtM(1) as:
1 - RR
ÄMtM(1) = N · TNE(t)
We then want to give a lower bound for ND(t) defined as the
number of defaults the structure can sustain before its reserve R(t) is fully
wiped out:
R(t)
?t ? [0,T], ND(t) : = ÄMtM(1)
R(t) TNE(t)
N
= 1-RR ·
= N
1 - RR(DR + SR(t) · D(t))
N
= 1-RR · DR
This last term does not depend on t. Assuming RR = 40%, N =
135 and DR = 1%, we find that ?t ? [0, T], ND(t) = 2.25. In other words, at any
point in time, the structure can stand 2 simultaneous defaults before breaking
the bond floor in the worst case scenario.
Conclusion
In this document, we have presented a theoretical modelling
framework for designing and rating a Dynamic Proportion Portfolio Insurance
(DPPI)) product, an innovation in the world of synthetic structured credits
providing a capital guarantee to the investor.
We first outline the main steps of Collateral Synthetic
Obligation risk-neutral pricing in the well-known one factor gaussian copula
model, which we further develop and supplement for rating DPPIs. However, the
latter requires to evaluate an expected loss function L(M) under the
historical risk measure: various discrete and continuous random processes are
introduced for describing risk factors such as defaults, rating transitions,
Credit Default Swap (CDS) spread levels and recovery rates. Process parametres
calibrated on historical data are assumed to be provided by Moody's, a rating
agency. We then rely upon a C++ implementation to run Monte-Carlo simulations
and estimate L(M).
The combination of several innovative investment rules such as
a dynamic leverage function, contingent coupon payments, the removal of
downgraded assets, lock-in and lock-out features, allows us to minimize
L(M), achieve the target rating of Aa3 in the base case scenario and
pass all required stress scenarios.
Finally, we describe the «gap-risk» hedging issue
faced by the investment bank when granting a capital guarantee to the investor.
We show that in a simplified framework, it can be measured through a
default-count equivalent function closely related to the DPPI's structural
features.
Appendix
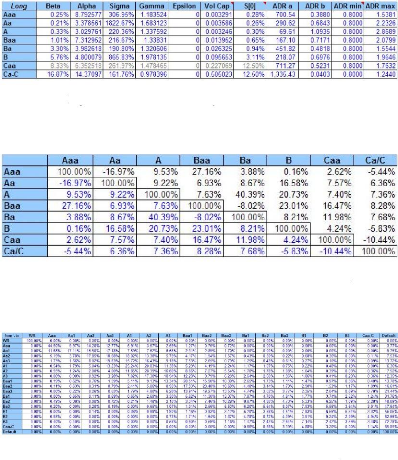
Figure 8: Parametres of Moody's CDS spread processes
Figure 9: Correlation matrix of Moody's CDS spread processes
Figure 10: Moody's 10Y corporate rating transition matrix
Moody's risk factors
Optimized DPPI parametres
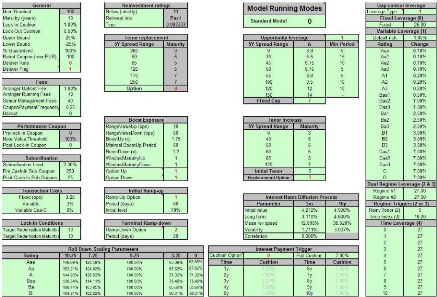
Figure 11: DPPI optimized structural features
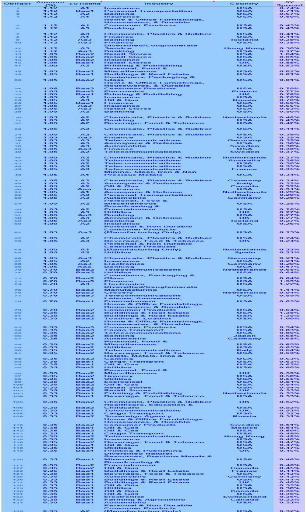
Figure 12: DPPI reference portfolio 41
Bibliography
[1] L. ANDERSEN, J. SIDENIUS & S. BASU, All your hedges in
one basket, RISK magazine, 2003
[2] J-P. LAURENT, J. GREGORY, Basket default swaps, CDOs and
factor copulas, Working paper, 2003
[3] D.X. LI, On default correlation: a copula factor approach,
The Journal of Fixed Income, 2000
[4] A. ELIZALDE, Credit Risk Models IV: understanding and
pricing CDOs, Working paper No. 0608, CEMFI, 2006
[5] Q. CHEN, CDO Pricing and Copula Method, Working paper,
University of Maryland, 2006
[6] A. LÜSCHER, Synthetic CDO pricing using the double
normal inverse Gaussian copula with stochastic factor loadings, Master's
thesis, ETH Zürich, 2005
[7] B. VERSCHUERE, On copulas and their application to CDO
pricing, Master's thesis, University Of Toronto, 2006
[8] M. VAN DER VOORT, Factor copulas: totally external defaults,
Working paper, ABN Amro, 2005
[9] E. PARCELL, Loss unit interpolation in the collateralized
debt obligation pricing model, Working paper, Derivative Fitch, 2006
[10] S. GALIANI, M. SHCHETKOVSKIY & A. KAKODKAR, Factor
models for synthetic CDO valuation, Internal working paper, Merrill Lynch,
2004
[11] M. SHCHETKOVSKIY et al, Base correlation and beyond,
Internal working paper, Merrill Lynch, 2006
[12] A. PAIN, O. RENAULT & D. SHELTON, Base correlation: the
term structure dimension, Internal working paper, Citigroup, 2005
[13] P. TEKLOS et al, CPDOs: the new best seller?, Special
report, Citigroup, 2006
[14] E. VARLOOT, CPDO: an asset class of its own or a glorified
bearish rated equity?, Special report, Union des Banques Suisses, 2006
[15] A. LINDEN et al, First Generation CPDO: case study on
performance and ratings, Special report, Derivative Fitch, 2007
[16] B. MARJOLIN et al, Moody's approach to modelling Exotic
synthetic CDOs with CDOROM, Special report, Moody's, 2007
[17] A. KAROLIK, Modeling Correlated Credit Rating Migrations,
PhD thesis, Carnegie Mellon University, 2006
[18] D. BUNIMOVICH, Modeling and Pricing of Collateralized Debt
Obligations, PhD thesis, Carnegie Mellon University, 2002
[19] V. DURRLEMANN, Les fonctions copules: définition,
examples et propriétés, Class notes, Ecole Nationale des Ponts et
Chaussées, 2006
[20] J-F. DELMAS, Mesures de risque, Class notes, Ecole
Nationale des Ponts et Chaussées, 2006
[21] P. MAZAUTAUD et al, Moody's Metric and Its Uses, Special
report, Moody's, 2006
[22] A. METZ, R. CANTOR, Introducing Moody's Credit Transition
Model, Special report, Moody's 2007
[23] A. METZ, R. CANTOR, A Cyclical Model of Multiple-Horizon
Credit Rating Transitions and Default, Special report, Moody's, 2007
[24] A. GUTTLER, Conditional Rating Transitions: The Case of
S&P and Moody's, European Business School, Working paper, 2007
[25] S. BALDER, M. BRANDL & A. MAHAYNI, Effectiveness of
CPPI Strategies under Discrete-Time Trading, University of Bonn, Working paper,
2005
|