3.1.2 Moody's Metric and coherent risk measures
We now temporarily put the risk-neutral measure aside and
focus on the historical probability measure. Moody's uses the same methodology
to rate CPDO and CPPI/DPPI products. It estimates the expected present value of
the loss function L(M) through Monte-Carlo simulations under the historical
probability measure.
Definition 2. Moody's expected discounted loss
Let t E I = {0, ät, .., kät, .., T} describe the
discrete time scale with T the maturity of the deal. Let Xt :=
(X(1)
t , .., X(p)
t ) be the p-vector of risk factors observed as of date t. We
introduce the filtration (Ft){t?I} defined as ?t E I, Ft := ó
(Xu,u E I,u = s). We further define the three stopping times (r, r,
r):
r := inf{s = 0, NPV (s) = TRV (s)}
r := inf{s = 0, NPV (s) = BF(s)} A T r := r A r
We then express Moody's risky discount factor DF(M) as
a function of the EURIBOR/LIBOR rate curve and of the senior spread s served to
the investor:
?t E I, DF(M)(t) :=
|
t-1Y i=0
|
1
|
1 + ät(EUR(iät, (i + 1)ät) + s)
|
>1p i=1 L(M) L(M) = i
p
|
+
|
ót99%
|
vp
|
Then, under the historical probability measure, Moody's expected
discounted loss L(M) is given below:
[ ]
L(M) := E 1{ô<ô} [A(1 - sl) - max(NP V
(r), BF (r)) + DI(r)]+ DF (M)(r)
where sl is the detachment point in % of the subordinated
note.
In practise, Moody's uses an unbiased empirical estimator of
L(M), L(M) defined as:
where t99% := Ö-1(99%), p is the number of
Monte-Carlo simulations, L(M)
i is the
loss calculatd on the ith scenario and
ó is the standard error of (L(M)
1 , .., L(M)
p ).
Moody's then maps that value L(M) and the maturity of the deal T
against a positive real scale S = [0, 21] through a function MM called
«Moody's Metric»:
MM : [0, 1] × R+ -? [0, 21]
(x,t) i-? MM(x,t)
We shall now describe how the Moody's Metric mapping function
works. We first define the letter-to-integer mapping function R:
R : {Aaa,Aa1,..,Ca,C} -? {1,..,21}
m -? R(m)
The discrete mapping table is given below:
Rating-Figure
|
Rating-Letter
|
1
|
Aaa
|
2
|
Aa1
|
3
|
Aa2
|
4
|
Aa3
|
5
|
A1
|
6
|
A2
|
7
|
A3
|
8
|
Baa1
|
9
|
Baa2
|
10
|
Baa3
|
11
|
Ba1
|
12
|
Ba2
|
13
|
Ba3
|
14
|
B1
|
15
|
B2
|
16
|
B3
|
17
|
Caa1
|
18
|
Caa2
|
19
|
Caa3
|
20
|
Ca
|
21
|
C
|
Figure 3.1: Moody's rating conversion table
We shall now define the discrete function EL that maps the
integer equivalent of a rating category and a maturity with a percentage
expected loss:
EL : {1, .., 21} × {1, .., T} -? [0, 1]
(m, t) '-? EL(m, t)
Moody's calibrates the function EL on historical default data by
using the cohort method.
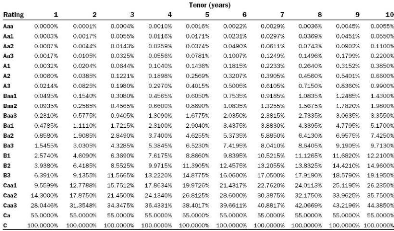
Figure 3.2: Moody's idealized EL values by rating category and
tenor
We shall then define EL, the time-continuous version
of EL function obtained by linearly interpolating EL between two discrete
integer dates:
EL : {1,..,21} x [0,T] -*[0,1]
(m, t) i-* (t + 1 - [t])EL(m, [t]) + (t - [t])EL(m, [t] + 1)
Let us now define the reverse mapping function F -1
that transforms any percentage loss level and tenor into a rating:
F -1 : [0, 1] x [0, T] -* {1, .., 21}
(x,t) -* min{m E {1,..,21}| EL(m,t) = x}
We finally give the expression of the Moody's Metric function MM:
Definition 3. Moody's Metric
?x E [0,1],?t E [0,T],
EL(F -1(x, t), t))
ln x - ln (
MM(x, t) := F -1(x, t) + ln ( EL(F
-1(x, t) + 1, t)) - ln ( EL(F-1(x, t), t))
In other words, the Moody's Metric can be seen as a
standardized continuous scale that allows to compare expected loss levels for
different tenors. We shall now take a closer look at the notion of risk measure
and understand to what extent it makes sense to use the expected loss as a
proxy for measuring risk.
Definition 4. Coherent Risk Measure
Let C denote a set of random variables representing all
possible risky positions and L E C be a random variable whose range of values
represents possible losses from any given risky position. We define the risk
measure function p as a mapping from C to R. The risk measure p is coherent if
it is:
i) monotonous: ?X, Y ? G, X = Y p(X) = p(Y )
ii) positively homogeneous: ?X ? G, ?h > 0, hX ? G and p(hX)
= hp(X)
iii) sub-additive: ?X, Y ? G, X + Y ? G and p(X + Y ) = p(X) +
p(Y )
iv) translation invariant: ?X ? G, ?a ? R s.t. X + a ? G, p(X +
a) = p(X) + a
Proposition 4.
If G+ is a set of non-negative random variables,
interpreted as a loss from a risky position, then expected value is a coherent
risk measure:
?X ? G+, p(X) := E[X]

Proof. Properties (i),(ii), (iii) and (iv) immediately result
from the expectation's linearity.
Unlike E[X], the Moody's Metric MM(X, t), where X is a
positive random variable that takes its values in [0, 1], is not a coherent
risk measure: though it is clearly monotonous and subadditive (because MM(., t)
is increasing and concave), it is neither positively homogeneous, nor
translation invariant.
|