VII. Annexes:
Modèle de la demande des services de
Mutuelles:
Bendroit(it) = f (txcot, insfem, inshom, wlinf, cotann,
convprest, tierpay) + uit . sum bendroit
Variable
|
Obs
|
Mean
|
Std. Dev.
|
Min
|
Max
|
bendroit
|
361
|
12089.58
|
85710.44
|
0
|
820295
|
. sum txcot
Variable
|
Obs
|
Mean
|
Std. Dev.
|
Min
|
Max
|
txcot
|
362
|
6873.959
|
29980.29
|
0
|
266712
|
. sum insfem
Variable
|
Obs
|
Mean
|
Std. Dev.
|
Min
|
Max
|
insfem
|
362
|
1699.729
|
18579.98
|
0
|
352862
|
. sum inshom
Variable
|
Obs
|
Mean
|
Std. Dev.
|
Min
|
Max
|
inshom
|
362
|
2147.82
|
27820.27
|
0
|
529293
|
. tis annee
. iis id
. xtreg bendroit txcot insfem inshom wlinf cotann
convprest tierpay, fe note: wlinf omitted because of collinearity
note: cotann omitted because of collinearity
note: convprest omitted because of
collinearity
Fixed-effects (within) regression Number of obs =
339
Group variable: id Number of groups = 216
R-sq: within = 0.6245 Obs per group: min = 1
between = 0.0001 avg = 1.6
overall = 0.0001 max = 2
F(4,119) = 49.48
corr(u_i, Xb) = -0.1169 Prob > F = 0.0000
bendroit
|
Coef
|
Std Err.
|
t
|
P>|t|
|
[95% Conf. Interval]
|
txcot
|
.0008849
|
.0051284
|
0.17
|
0.863
|
-.0092698 .0110397
|
insfem
|
-3198637
|
.1012899
|
-3.16
|
0.002
|
-.5204279 -.1192995
|
inshom
|
.5508696
|
.0824549
|
6.68
|
0.000
|
.3876006 .7141387
|
wlinf
|
(omitted) (omitted) (omitted)
|
cotann
|
convprest
|
tierpay
|
541.2878
|
59.40769
|
9.11
|
0.000
|
423.6546 658.9209
|
_cons
|
11647.37
|
93.90184
|
124.04
|
0.000
|
11461.44 11833.31
|
sigma_u sigma_e rho
|
83185.228 39.517235 .99999977 (fraction of variance
due to u_i)
|
F test that all u_i=0: F(215, 119) = 6.4e+06 Prob > F
= 0.0000
. estimates store fixed
. xtreg bendroit txcot insfem inshom wlinf cotann
convprest tierpay, re Random-effects GLS regression Number of obs =
339
Group variable: id Number of groups = 216
R-sq: within = 0.6229 Obs per group: min = 1
Between = 0.0967 avg = 1.6
overall = 0.1137 max = 2
Random effects u_i ~ Gaussian Wald chi2 (7) =
207.53
corr(u_i, X) = 0 (assumed) Prob > chi2 =
0.0000
bendroit
|
Coef
|
Std Err.
|
z
|
P>|z|
|
[95% Conf. Interval]
|
txcot
|
.0007184
|
.0052836
|
0.14
|
0.892
|
-.0096373 .0110741
|
insfem
|
-3066109
|
.1042986
|
-2.94
|
0.003
|
-.5110325 -.1021893
|
inshom
|
.5087155
|
.08406
|
6.05
|
0.000
|
.343961 .6734701
|
wlinf
|
-6374.74
|
17786.14
|
-4.29
|
0.000
|
-111234.9 -41514.56
|
cotann
|
-2566.09
|
12376.08
|
-1.02
|
0.310
|
-36822.76 11690.58
|
convprest
|
4894.395
|
30339.13
|
0.16
|
0.872
|
-54569.21 64358
|
tierpay
|
550.6772
|
61.18269
|
9.00
|
0.000
|
430.7613 670.593
|
_cons
|
81272.4
|
31044.56
|
2.62
|
0.009
|
20426.18 142118.6
|
sigma_u
|
75429.219
|
sigma_e
|
39.517235
|
rho
|
.99999973 (fraction of variance due to u_i)
|
. hausman fixed
|
---- Coefficients ----
|
|
|
|
(b) (B)
|
(b-B)
|
sqrt(diag(V_b-V_B))
|
|
fixed .
|
Difference
|
S.E.
|
Txcot
|
.0008849 .0007184
|
.0001665
|
-
|
Insfem
|
-.3198637 -.3066109
|
-.0132528
|
-
|
Inshom
|
.5508696 .5087155
|
.0421541
|
-
|
tierpay
|
541.2878 550.6772
|
-9.389402
|
-
|
b = consistent under Ho and Ha; obtained from xtreg B =
inconsistent under Ha, efficient under Ho; obtained from xtreg Test: Ho:
difference in coefficients not systematic
chi2(4) = (b-B)'[(V_b-V_B)^(-1)](b-B)
= 244.30
Prob>chi2 = 0.0000
(V_b-V_B is not positive definite)
. regress bendroit txcot insfem inshom wlinf cotann
convprest tierpa
Source
|
SS df MS
|
Number of obs = 339 F( 7, 331) = 11.06
Prob > F = 0.0000
R-squared = 0.1896
Adj R-squared = 0.1725 Root MSE = 80419
|
Model
|
5.0080e+11 7 7.1543e+10
|
Residual
|
2.1407e+12 331 6.4673e+09
|
Total
|
2.6415e+12 338 7.8150e+09
|
bendroit
|
Coef
|
Std Err.
|
z
|
P>|z|
|
[95% Conf. Interval]
|
txcot
|
-1971859
|
.1620467
|
-1.22
|
0.225
|
-.5159572 .1215855
|
insfem
|
-4449882
|
6.194993
|
-0.07
|
0.943
|
-12.63151 11.74153
|
inshom
|
.0976121
|
4.130537
|
0.02
|
0.981
|
-8.027802 8.223026
|
wlinf
|
-16161.5
|
16049.43
|
-7.24
|
0.000
|
-147733.3 -84589.76
|
cotann
|
-5855.04
|
10321.5
|
-2.50
|
0.013
|
-46159.05 -5551.033
|
convprest
|
18084.64
|
23465.62
|
0.77
|
0.441
|
-28075.91 64245.18
|
tierpay
|
48390.69
|
13173.49
|
3.67
|
0.000
|
22476.37 74305
|
_cons
|
77104.98
|
25087.07
|
3.07
|
0.002
|
27754.77 126455.2
|
. predict residu4, resid
(23 missing values generated) . gen residu5 = residu4^2
(23 missing values generated)
. regress residu5 txcot insfem inshom wlinf cotann
convprest tierpay
Source
|
SS df MS
|
Number of obs = 339
F( 7, 331) = 10.79
Prob > F = 0.0000
R-squared = 0.1858
Adj R-squared = 0.1686 Root MSE = 4.0e+10
|
Model
|
1.2107e+23 7 1.7296e+22
|
Residual
|
5.3062e+23 331 1.6031e+21
|
Total
|
6.5169e+23 338 1.9281e+21
|
bendroit
|
Coef
|
Std Err.
|
t
|
P>|t|
|
[95% Conf. Interval]
|
txcot
|
-14306.1
|
80678.16
|
-1.42
|
0.157
|
-273012.7 44400.5
|
insfem
|
-21744.3
|
3084299
|
-0.20
|
0.840
|
-6689044 5445556
|
inshom
|
308706.3
|
2056469
|
0.15
|
0.881
|
-3736691 4354103
|
wlinf
|
-.96e+10
|
7.99e+09
|
-7.46
|
0.000
|
-7.53e+10 -4.39e+10
|
cotann
|
-.09e+10
|
5.14e+09
|
-2.12
|
0.035
|
-2.10e+10 -7.1e+08
|
convprest
|
2.34e+10
|
1.17e+10
|
2.00
|
0.046
|
4.15e+08 4.64e+10
|
tierpay
|
2.03e+10
|
6.56e+09
|
3.10
|
0.002
|
7.45e+09 3.32e+10
|
_cons
|
2.80e+10
|
1.25e+10
|
2.24
|
0.026
|
3.43e+09 5.26e+10
|
. regress bendroit txcot insfem inshom wlinf cotann
convprest tierpay, robust Linear regression Number of obs = 339
F( 7, 331) = 1.75
Prob > F
|
=
|
0.0972
|
R-squared
|
=
|
0.1896
|
Root MSE
|
=
|
80419
|
bendroit
|
Coef.
|
Robust Std. Err.
|
t
|
P>|t|
|
[95% Conf. Interval]
|
txcot
|
-.1971859
|
.1671438
|
-1.18
|
0.239
|
-.525984 .1316122
|
insfem
|
-.4449882
|
4.96963
|
-0.09
|
0.929
|
-10.22103 9.331053
|
inshom
|
.0976121
|
3.300771
|
0.03
|
0.976
|
-6.395522 6.590746
|
wlinf
|
-116161.5
|
43435.85
|
-2.67
|
0.008
|
-201606.6 -30716.38
|
cotann
|
-25855.04
|
9949.651
|
-2.60
|
0.010
|
-45427.57 -6282.52
|
convprest
|
18084.64
|
24978.26
|
0.72
|
0.470
|
-31051.52 67220.79
|
tierpay
|
48390.69
|
18627.91
|
2.60
|
0.010
|
11746.67 85034.7
|
_cons
|
77104.98
|
25509.76
|
3.02
|
0.003
|
26923.29 127286.7
|
. ovtest
Ramsey RESET test using powers of the fitted values of
bendroit Ho: model has no omitted variables
F(3, 328) = 55.48
Prob > F = 0.0000
Number of obs
|
|
= 339
|
F( 7, 331)
|
=
|
11.06
|
Prob > F
|
=
|
0.0000
|
R-squared
|
=
|
0.1896
|
Adj R-squared
|
=
|
0.1725
|
Root MSE
|
=
|
80419
|
. regress bendroit txcot insfem inshom wlinf cotann
convprest tierpa
Source
|
SS
|
df
|
MS
|
Model
|
5.0080e+11
|
7
|
7.1543e+10
|
Residual
|
2.1407e+12
|
331
|
6.4673e+09
|
Total
|
2.6415e+12
|
338
|
7.8150e+09
|
Bendroit
|
Coef.
|
Robust Std. Err.
|
t
|
P>|t|
|
[95% Conf. Interval]
|
txcot
|
-.1971859
|
.1620467
|
-1.22
|
0.225
|
-.5159572 .1215855
|
insfem
|
-.4449882
|
6.194993
|
-0.07
|
0.943
|
-12.63151 11.74153
|
inshom
|
.0976121
|
4.130537
|
0.02
|
0.981
|
-8.027802 8.223026
|
wlinf
|
-116161.5
|
16049.43
|
-7.24
|
0.000
|
-147733.3 -84589.76
|
cotann
|
-25855.04
|
10321.5
|
-2.50
|
0.013
|
-46159.05 -5551.033
|
convprest
|
18084.64
|
23465.62
|
0.77
|
0.441
|
-28075.91 64245.18
|
tierpay
|
48390.69
|
13173.49
|
3.67
|
0.000
|
22476.37 74305
|
_cons
|
77104.98
|
25087.07
|
3.07
|
0.002
|
27754.77 126455.2
|
. predict residu6, resid
(23 missing values generated)
. gen rresidu6 = residu6[_ n-1]
(24 missing values generated)
. regress residu6 rresidu6 txcot insfem inshom wlinf
cotann convprest tierpa
Number of obs
|
|
= 320
|
F( 7, 331)
|
=
|
18.63
|
Prob > F
|
=
|
0.0000
|
R-squared
|
=
|
0.3239
|
Adj R-squared
|
=
|
0.3066
|
Root MSE
|
=
|
65356
|
Source
|
SS
|
df
|
MS
|
Model
|
6.3652e+11
|
8
|
7.9565e+10
|
Residual
|
1.3284e+12
|
311
|
4.2714e+09
|
Total
|
1.9649e+12
|
319
|
6.1596e+09
|
residu6 | Coef. Std. Err.
+
rresidu6| .5777769 .0498382
txcot | .1063601 .1345919
insfem| 1.936321 6.854951
inshom| -10.33042 7.738755
wlinf | -11670.37 14181.53
cotann | 6432.463 8856.809 convprest | -2410.942
19787.15
tierpay | -388.4665 11246.15
_cons | 13372.52 21877.29
t
|
P>|t|
|
[95% Conf. Interval]
|
11.59
|
0.000
|
.4797141
|
.6758397
|
0.79
|
0.430
|
-.1584658
|
.371186
|
0.28
|
0.778
|
-11.55163
|
15.42427
|
-1.33
|
0.183
|
-25.55736
|
4.896515
|
-0.82
|
0.411
|
-39574.25
|
16233.52
|
0.73
|
0.468
|
-10994.38
|
23859.31
|
-0.12
|
0.903
|
-41344.55
|
36522.67
|
-0.03
|
0.972
|
-22516.64
|
21739.71
|
0.61
|
0.541
|
-29673.71
|
56418.75
|
. prais bendroit txcot insfem ins hom wlinf
cotann convprest tierpay , robust
Number of gaps in sample: 215 (gap count
includes panel changes)
(note: computations for rho restarted at each
gap)
1teration 0: rho = 0.0000
1teration 1: rho = 0.9974 1teration
2: rho = 0.9999 1teration 3:
rho = 1.0000 1teration 4: rho =
1.0000 1teration 5: rho =
1.0000
1teration 6: rho = 1.0000
Prais-Winsten AR(1) regression
-- iterated estimates Linear regression
Number of obs = F( 8, 331) =
Prob > F =
R-squared =
Root MSE =
|
339 92.51 0.0000
0.1413 260.83
|
Semirobust
Std. Err.
|
t
|
P>Iti
|
[95% Conf. 1nterval]
|
.0079714
|
-0.81
|
0.416
|
-.0221733
|
.0091888
|
.396713
|
-0.53
|
0.597
|
-.990221
|
.5705723
|
.3403639
|
0.33
|
0.741
|
-.5570809
|
.7820175
|
43691.54
|
-1.92
|
0.056
|
-169662
|
2234.228
|
7716.224
|
-1.78
|
0.076
|
-28901.62
|
1456.425
|
29619.47
|
0.28
|
0.780
|
-49992.26
|
66540
|
76.01712
|
8.73
|
0.000
|
514.352
|
813.4272
|
38022.54
|
2.27
|
0.024
|
11452.35
|
161045
|
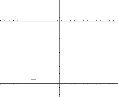
Coef.
bendroit
txcot
-.0064923
insfem
ins hom
-.2098243
.1124683
wlinf
cotann
-83713.87
- 13722.6
8273.87
663.8896
convprest tierpay
!cons
rho
86248.65
. 9999913
Durbin-Watson statistic (original)
0.001063
Durbin-Watson statistic (transformed)
0.811749
Modèle de l'offre des Mutuelles: . sum
txrecouv
Variable
|
Obs
|
Mean
|
Std. Dev.
|
Min
|
Max
|
txrecouv
|
362
|
1.695746
|
9.10259
|
0
|
100
|
. sum depmal
Variable
|
Obs
|
Mean
|
Std. Dev.
|
Min
|
Max
|
depmal
|
362
|
1.27e+07
|
7.99e+07
|
0
|
9.00e+08
|
. sum payech
Variable
|
Obs
|
Mean
|
Std. Dev.
|
Min
|
Max
|
payech
|
342
|
.7453216
|
.3761493
|
0
|
1.4
|
. xtreg txrecouv depmal logges rur payech partorg,
fe
note: logges omitted because of collinearity
note: rur omitted because of collinearity
Fixed-effects (within) regression Number of obs =
320
Obs per group: min =
|
1
|
avg =
|
1.5
|
max =
|
2
|
Group variable: id Number of groups = 207 R-sq: within
= 0.8276
between = 0.0451
overall = 0.0233
corr(u_i, Xb) = -0.3999
txrecouv | Coef.
+
|
Std. Err.
|
t
|
P>|t|
|
F(3,110) = 176.01
Prob > F = 0.0000
[95% Conf. Interval]
|
depmal | -1.67e-08
|
3.97e-08
|
-0.42
|
0.675
|
-9.55e-08
|
6.21e-08
|
logges | (omitted)
|
|
|
|
|
|
rur | (omitted)
|
|
|
|
|
|
payech | .9602815
|
.0429814
|
22.34
|
0.000
|
.8751024
|
1.045461
|
partorg | .9412221
|
.0467686
|
20.13
|
0.000
|
.8485377
|
1.033907
|
_cons | -.2253838
|
.5410685
|
-0.42
|
0.678
|
-1.297655
|
.846887
|
+
sigma_u | 7.365344
sigma_e | .04663564
rho | .99995991 (fraction of variance due to
u_i)
F test that all u_i=0: F(206, 110) = 21165.05 Prob > F
= 0.0000
. estimates store fixed
. xtreg txrecouv depmal logges rur payech partorg,
re
Number of obs =
|
320
|
Number of groups =
|
207
|
Obs per group: min =
|
1
|
avg =
|
1.5
|
max =
|
2
|
Random-effects GLS regression Group variable:
id
R-sq: within = 0.8263
Between = 0.0458
overall l = 0.0269
Random effects u_i ~ Gaussian Wald chi2(5) =
524.29
corr(u_i, X) = 0 (assumed) Prob > chi2 =
0.0000
txrecouv | Coef. Std. Err.
+ depmal | 1.90e-08 5.92e-09
logges | .2620777 1.029565
rur | .3375233 1.125442 payech | .9547098 .0432859
partorg | .9370785 .0472341
_cons | -.9711391 1.147288 + sigma_u |
6.6806046
sigma_e | .04663564
z
|
P>|z|
|
[95% Conf. Interval]
|
3.21
|
0.001
|
7.39e-09
|
3.06e-08
|
0.25
|
0.799
|
-1.755832
|
2.279987
|
0.30
|
0.764
|
-1.868302
|
2.543349
|
22.06
|
0.000
|
.869871
|
1.039549
|
19.84
|
0.000
|
.8445014
|
1.029656
|
-0.85
|
0.397
|
-3.219783
|
1.277505
|
rho | .99995127 (fraction of variance due to
u_i)
. hausman fixed
Note: the rank of the differenced variance matrix (2)
does not equal the number of coefficients being tested (3); be sure this is
what you expect, or there may
be problems computing the test. Examine the output of
your estimators for anything unexpected and possibly consider scaling your
variables so that the coefficients are on a similar scale.
---- Coefficients ----
| (b) (B) (b-B) sqrt(diag(V_b-V_B))
| fixed . Difference S.E.
+
depmal | -1.67e-08 1.90e-08 -3.57e-08
3.93e-08
payech | .9602815 .9547098 .0055717 .
partorg | .9412221 .9370785 .0041436 .
b = consistent under Ho and Ha; obtained from
xtreg
B = inconsistent under Ha, efficient under Ho; obtained
from xtreg
Test: Ho: difference in coefficients not
systematic
chi2(2) = (b-B)'[(V_b-V_B)^(-1)](b-B)
= 13.13
Prob>chi2 = 0.0014
(V_b-V_B is not positive definite)
. regress txrecouv depmal logges rur payech
partorg
Source | SS df MS Number of obs = 320
+ F( 5, 314) = 2.70
Model | 407.42024 5 81.484048 Prob > F =
0.0210
Residual | 9482.72946 314 30.1997754 R-squared =
0.0412
+ Adj R-squared = 0.0259
Total | 9890.1497 319 31.0036041 Root MSE =
5.4954
txrecouv | Coef. Std. Err. t P>|t| [95% Conf.
Interval]
+
depmal | 1.06e-08 3.69e-09 2.86 0.005 3.29e-09
1.78e-08 logges | .1823536 .6830243 0.27 0.790 -1.161529
1.526236
rur | .1880172 .757224 0.25 0.804 -1.301857 1.677892
payech | -1.000854 .8247842 -1.21 0.226 -2.623657 .6219481 partorg | 1.357199
.8401453 1.62 0.107 -.2958265 3.010225 _cons | .324838 1.117904 0.29 0.772
-1.874692 2.524368
. predict residu10, resid
(42 missing values generated) . summarize residu10,
detail
1% 5%
|
Residuals
Percentiles Smallest
-4.283229 -10.19489
-1.953269 -10.19489
|
|
10%
|
-1.264102
|
-4.933321
|
Obs 320
|
25%
|
-.7451477
|
-4.283229
|
Sum of Wgt. 320
|
50%
|
.0999992
|
|
Mean -9.37e-09
|
|
|
Largest
|
Std. Dev. 5.452194
|
75%
|
.2012891
|
1.285645
|
|
90%
|
.4724982
|
1.305645
|
Variance 29.72642
|
95%
|
.6400636
|
1.307613
|
Skewness 16.62632
|
99%
|
1.285645
|
95.12834
|
Kurtosis 291.5051
|
. gen residu11 = residu10^2 (42 missing values
generated)
. regress residu11 depmal logges rur payech
partorg
Source | SS
+
Model | 3811003.62 Residual | 77822877.4 +
Total | 81633881
df
|
MS
|
Number of obs = F( 5, 314) =
|
320
3.08
|
5
|
762200.723
|
Prob > F
|
=
|
0.0100
|
314
|
247843.559
|
R-squared
|
=
|
0.0467
|
|
|
Adj R-squared
|
=
|
0.0315
|
319
|
255905.583
|
Root MSE
|
=
|
497.84
|
residu11 | Coef. Std. Err. t P>|t| [95% Conf.
Interval]
1.04e-06
|
3.35e-07
|
3.11
|
0.002
|
3.81e-07
|
1.70e-06
|
27.43666
|
61.87612
|
0.44
|
0.658
|
-94.30757
|
149.1809
|
11.13803
|
68.59798
|
0.16
|
0.871
|
-123.8318
|
146.1078
|
-123.7671
|
74.71836
|
-1.66
|
0.099
|
-270.779
|
23.24485
|
77.97637
|
76.10993
|
1.02
|
0.306
|
-71.77356
|
227.7263
|
29.64055
|
101.2725
|
0.29
|
0.770
|
-169.618
|
228.8991
|
+ depmal | logges |
rur | payech | partorg | _cons |
. regress txrecouv depmal logges rur payech partorg,
robust
Linear regression
Number of obs =
320
|
F( 5, 314)
|
=
|
5.36
|
Prob > F
|
=
|
0.0001
|
R-squared
|
=
|
0.0412
|
Root MSE
|
=
|
5.4954
|
|
|
txrecouv | Coef.
+ depmal | 1.06e-08 logges | .1823536
rur | .1880172 payech | -1.000854 partorg | 1.357199
_cons | .324838
Robust Std. Err.
t
|
P>|t|
|
[95% Conf. Interval]
|
1.16e-08
|
0.91
|
0.364
|
-1.23e-08
|
3.34e-08
|
.3079377
|
0.59
|
0.554
|
-.4235285
|
.7882357
|
.146811
|
1.28
|
0.201
|
-.1008405
|
.4768749
|
1.305616
|
-0.77
|
0.444
|
-3.569716
|
1.568007
|
.8632804
|
1.57
|
0.117
|
-.3413459
|
3.055745
|
.3857792
|
0.84
|
0.400
|
-.434201
|
1.083877
|
|
. ovtest
Ramsey RESET test using powers of the fitted values of txrecouv
Ho: model has no omitted variables
F(3, 311) = 24.66
Prob > F = 0.0000
. regress txrecouv depmal logges rur payech
partorg
Source | SS df MS Number of obs = 320
+ F( 5, 314) = 2.70
Model | 407.42024 5 81.484048 Prob > F =
0.0210
Residual | 9482.72946 314 30.1997754 R-squared =
0.0412
+ Adj R-squared = 0.0259
Total | 9890.1497 319 31.0036041 Root MSE =
5.4954
txrecouv | Coef. Std. Err.
+ depmal | 1.06e-08 3.69e-09 logges | .1823536
.6830243
rur | .1880172 .757224 payech | -1.000854 .8247842
partorg | 1.357199 .8401453 _cons | .324838 1.117904
t
P>|t|
|
[95% Conf. Interval]
|
2.86
|
0.005
|
3.29e-09
|
1.78e-08
|
0.27
|
0.790
|
-1.161529
|
1.526236
|
0.25
|
0.804
|
-1.301857
|
1.677892
|
-1.21
|
0.226
|
-2.623657
|
.6219481
|
1.62
|
0.107
|
-.2958265
|
3.010225
|
0.29
|
0.772
|
-1.874692
|
2.524368
|
|
. predict residu12, resid
(42 missing values generated)
. gen rresidu12 = residu12[_n-1]
(43 missing values generated)
. regress residu12 rresidu12 depmal logges rur payech
partorg
Source | SS
|
df
|
MS
|
Number of obs = 297
|
+
|
|
|
F( 6, 290)
|
= 0.01
|
Model | 1.47375498
|
6
|
.24562583
|
Prob > F
|
= 1.0000
|
Residual | 9467.7714
|
290
|
32.6474876
|
R-squared
|
= 0.0002
|
+
|
|
|
Adj R-squared = -0.0205
|
Total | 9469.24515
|
296
|
31.9906931
|
Root MSE
|
= 5.7138
|
residu12 | Coef.
|
Std. Err. t P>|t|
|
[95% Conf. Interval]
|
|
|
+
rresidu12 | .0053267 .0598405 0.09 0.929 -.11245
.1231033 depmal | -5.98e-11 3.86e-09 -0.02 0.988 -7.65e-09 7.53e-09 logges |
.0369564 .7466474 0.05 0.961 -1.432578 1.506491
rur | -.0163634 .8398714 -0.02 0.984 -1.66938
1.636653 payech | -.1283227 .9005571 -0.14 0.887 -1.900779 1.644134 partorg |
.1069494 .9181869 0.12 0.907 -1.700206 1.914105 _cons | .0351771 1.232258 0.03
0.977 -2.390125 2.46048
. prais txrecouv depmal logges rur payec h
partorg, robust
Number of gaps in sample: 206 (gap count includes panel
changes)
(note: computations for rho restarted at each gap)
1teration 0: rho = 0.0000 1teration 1: rho =
0.4416 1teration 2: rho = 0.7638 1teration 3:
rho = 0.9290 1teration 4: rho = 0.9828
1teration 5: rho = 0.9953 1teration 6: rho =
0.9982 1teration 7: rho = 0.9988 1teration 8:
rho = 0.9990 1teration 9: rho = 0.9990
1teration 10: rho = 0.9990 1teration 11: rho =
0.9990 1teration 12: rho = 0.9990
Prais-Winsten AR(1) regression
-- iterated estimates Linear regression
Number of obs
=
|
320
|
F( 6, 314)
|
=
|
129.68
|
Prob > F
|
=
|
0.0000
|
R-squared
|
=
|
0.1409
|
Root MSE
|
=
|
.2442
|
|
Semirobust
Coef. Std.
Err.
1.97e-08
2.24e-08
.2470148 .3376503
. 3318951 .3011336
. 9173285 .046247
. 9000429 .057686
-.9149577 .2316476
. 9990306
t
|
P>ItI
|
[95% Conf.
|
1nterval]
|
0.88
|
0.379
|
-2.43e-08
|
6.37e-08
|
0.73
|
0.465
|
-.4173283
|
.9113578
|
1.10
|
0.271
|
-.2605997
|
.9243899
|
19.84
|
0.000
|
.8263353
|
1.008322
|
15.60
|
0.000
|
.7865428
|
1.013543
|
-3.95
|
0.000
|
-1.370735
|
-.4591799
|
txrecouv
depmal logges
rur
payec partorg _cons
rho
Durbin-Watson statistic (original)
0.018083
Durbin-Watson statistic (transformed)
0.069279
. regress bendroit txcot insfem ins hom wlinf
cotann convprest tierpay
Estimateur WITHIN pour la demande : transformation
Frish-Waugh-Lovel :
. xtdata bendroit txcot insfem ins hom wlinf
cotann convprest tierpay, fe
note: wlinf omitted because of collinearity note: cotann
omitted because of collinearity note: convprest omitted because of
collinearity
SS df MS
309068.185 4 77267.0462
185831.815 334 556.382681
494900 338 1464.20118
Number of obs
|
=
|
339
|
F( 4, 334)
|
=
|
138.87
|
Prob > F
|
=
|
0.0000
|
R-squared
|
=
|
0.6245
|
Adj R-squared
|
=
|
0.6200
|
Root MSE
|
=
|
23.588
|
Model Residual
Source
Total
Coef. Std.
Err. t
. 0008849 .0030611 0.29
-.3198637 .0604598
-5.29
. 5508696 .0492172
11.19 (omitted)
(omitted)
(omitted)
541.2878 35.46034
15.26
11647.37 56.04984
207.80
P>ItI
|
[95% Conf. Interval]
|
0.773
|
-.0051366
|
.0069064
|
0.000
|
-.4387936
|
-.2009337
|
0.000
|
.4540549
|
.6476844
|
0.000
|
471.534
|
611.0415
|
0.000
|
11537.12
|
11757.63
|
|
bendroit
txcot insfem ins
hom wlinf cotann convprest tierpay _cons
. predict residu1, resid
. summarize residu1, detail
Residuals
Percentiles Smallest
1% -67.48943
-238.7236
5% -.9733983
-117.2276
10% 1.82e-12
-84.80728
25% 1.82e-12
-67.48943
50% 1.82e-12
Largest
75% 1.82e-12
67.48943
90% 1.82e-12
84.80728
95% .9733983 117.2276
99% 67.48943 238.7236
Obs
|
339
|
Sum of Wgt.
|
339
|
Mean
|
1.59e-12
|
Std. Dev.
|
23.44778
|
variance
|
549.7983
|
Skewness
|
-2.04e-13
|
Kurtosis
|
69.48069
|
|
Estimateur WITHIN pour l'offre :
. xtdata txrecouv depmal logges rur payec h
partorg, fe
. regress txrecouv depmal logges rur partorg
note: logges omitted because of collinearity note: rur omitted because of
collinearity
SS df MS
.062809628 2 .031404814
1.32484037 323 .004101673
1.38764999 325 .004269692
Number of obs
|
=
|
326
|
F( 2, 323)
|
=
|
7.66
|
Prob > F
|
=
|
0.0006
|
R-squared
|
=
|
0.0453
|
Adj R-squared
|
=
|
0.0394
|
Root MSE
|
=
|
.06404
|
|
Model Residual
Source
Total
txrecouv
Coef. Std.
Err. t P>ItI [95% Conf. Interval]
6.36e-08
5.44e-08
(omitted) (omitted)
-.0628895 .0177685
. 0527019 .7305845
1.17
|
0.243
|
-3.54
|
0.000
|
0.07
|
0.943
|
-4.33e-08
|
1.71e-07
|
-.097846
|
-.0279329
|
-1.384603
|
1.490007
|
depmal logges rur partorg _cons
.
1% -.2880782 -.3805699
5% -.0274953 -.3786813
10% -.020285 -.3541501
25% -.0052701 -.2880782
50% 0
Largest
75% .0052701 .2880782
90% .020285 .3541501
95% .0274953 .3786813
99% .2880782 .3805699
Obs
|
326
|
Sum of Wgt.
|
326
|
Mean
|
0
|
Std. Dev.
|
.0638469
|
variance
|
.0040764
|
Skewness
|
0
|
Kurtosis
|
26.57714
|
predict residu1, resid
. sum residu1, detail
Residuals
Percentiles Smallest
|