Article 3 :
ANALYTIC IMPORTANCE OF THE CODING FEATURES FOR
THE DISCRIMINATION OF VOWELS IN THE COCHLEAR IMPLANT SIGNAL C.
Berger-Vachon, S. Gallego, A. Morgon, E. Truy
An Otol Rhinol Laryngol 1995, sup.166,104: 9,2, 351-353
L'objectif de cette étude est de modéliser, en
utilisant la logique flou, la reconnaissance des voyelles /a/, /i/, /u/, /3/ de
4 sujets porteurs d'un implant cochléaire Nucleus de Cochlear.
Différents modèles de reconnaissance n'utilisant qu'une partie de
l'information transmise au patient via l'implant cochléaire sont
expérimentés (255 modèles).
10 locuteurs (5 hommes et 5 femmes) ont été
enregistrés. 48 items par locuteur ont été
prononcés. Chaque item est présenté en parallèle au
sujet implanté cochléaire et via l'implant cochléaire
à une carte d'acquisition permettant la reconnaissance par
ordinateur.
Pour chaque patient, une comparaison entre la matrice de
confusion et celle trouvée par ordinateur détermine le meilleur
modèle caractérisant sa reconnaissance.
Les résultats montrent que le modèle
représentant le mieux la compréhension varie selon le patient.
Certains sujets utilisent la tonotopie, d'autres n'utilisent que
l'énergie.
Il est donc tout aussi important pour certain sujet de
préserver l'enveloppe temporelle du signal que l'information
fréquentielle.
ANALYTIC IMPORTANCE OF THE CODING FEATURES FOR THE DISCRIMINATION
OF VOWELS IN THE COCHLEAR IMPLANT SIGNAL
C. BERGER-VACHON, MD, PIO; S. GALLEGO, MS; A. MORGON, MD; E.
TRUY, MD
From the Department of Otorhinoleryngology, Edouard I Terriot
Hospital, Lyon, France.
The présent study considers the analytic importance of
the excitation pulse features delivered by a Nucleus cochlear implant using the
FOF 1 F2 strategy. Four cochlear implant patients and 10 speakers uttering two
48-item lists constructed from four basic French vowels participatcd in this
study. Patterns were presented to the patients and played at the input of an
acquisition system in order to record the pulse features. Confusion matrices
obtained with the patients and with automatic recognition procedures were then
compared in order to find out the best-matching models simulating the patients'
performances, out of 255 possibilitics. The automatic recognition was carried
out according to fuzzy logic based on the elementary features of the pulses
coding the vowels. Results show that the essential features strongly depended
on the subject.
INTRODUCTION
Coding of the acoustic signal by means of a cochlear implant
(CI) is still under discussion.1 While several strategies have bcen
used, results based only on cl inical performances do not show with enough
precision what elementary features are important in the coding for speech
recognition. Basically, the stratégies commonly used in a multichannel
CI involve the phonetic aspect of language (Nucleus),2 the use of a
spectrum (Digisonic),3 and the analog splitting up of energy such as
the one produced by Symbion.4 Another step takes place in
experiments in which the signal is artificially modified and presented to the
patient in order to establish whether or not changes in the coding are
significant.5-7
Preliminary experiments have shown that on some pa-
Duration (D) Duration (D)
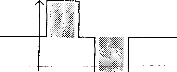
Amplitude (A)
Ne ·
Charge C=AxD
Fig I. Structure and parameters of stimulating pulse on any given
electrode (E, in text).
) )
tients, vowels with high energy are not confused with
lowenergy vowels, even when their frequency configuration is similar. For
example, the high energy of the French vowel /a/ makes it quite easy to
recognize. The question that emcrged from this finding is the following: "What
characteristics in the signal coded by the speech processor allow for the
acoustic distinction by the patient?"
Computer simulation allows the testing of a large
number of combinations of elementary signal features with the help of models
for determining the most important features for the patient. The study
presented here aims to find out what is
TABLE 1. MOST LIKELY VALUES FOR FEATURES ESTABLISIIED DURING
LEARNING STAGE (CORRESPONDING TO ONE SPEAKER AND ONE PATIENT)
|
/a/
|
li/
|
lu/
|
le/
|
El
|
19
|
21
|
21
|
20
|
E2
|
16
|
9
|
18
|
16
|
Al
|
11
|
22
|
26
|
5
|
A2
|
24
|
11
|
20
|
13
|
Dl
|
26
|
7
|
8
|
24
|
D2
|
17
|
29
|
6
|
15
|
Cl
|
29
|
4
|
4
|
26
|
C2
|
27
|
30
|
2
|
25
|
Units are artificiel numbers calculated from confusion matrix.
|
Magnetic
|
CI
|
Acquisitior
|
Desktop
|
|
Tape
|
_ Speech
|
|
|
-- Storage
|
Recorder
|
Processor
|
Caïd
|
Computer
|
|
Fig 2. Block diagrarn of acquisition system.
important in the coding that allows distinction between vowels.
Some work already done by the Melbourne team8 established that the
second formant (F2) and the F1F2 representation were important in the patient's
recognition process. This testing could be extended by using theoretic models.
A more analytic study of the features of the stimulation pulse is also
possible. Models can be created to evaluate the contribution of each feature of
those elements that play a role in the distinction perceived by the patient.
This is the aim of our work.
PA VENTS
The four patients who collaborated in this work all used the
Nucleus 22 cochlear implant, and the Mini Speech Processor (MSP) programmed
with the FOF1F2 strategy and in the bipolar plus one mode. They were two women,
one man, and a young girl. The FOF1F2 strategy was chosen in order to limit the
number of features studied in this experiment.
The man (CO), 46 years of age, had all his electrodes working.
He became deaf at the age of 41. He had 20 channels active and was a star
patient. The first woman (BA), 40 years of age, also had all her electrodes
working and 20 channels active. Deafness occurred at the age of 2. The second
woman (LA), 32 ycars old, had an ossificd cochlea with only 5 channels active
(15 to 20; 18 was nonfunctioning). She became deaf at the age of 28. The girl
(AM), 12 years old, had 19 channels active (channel 7 was closed). She was
close to being a star patient. Deafness occurred at the age of 9.
In all four cases, the channels covered the frequency range
from 280 Hz to 4 kHz; two fifths were in the Fl range (280 to 800 Hz) and the
others were in the F2-F3 range (800 to 4,000 Hz). The band-pass filter was
distributed according to a logarithmic scale.
ACOUSTIC MATERIAL
Speakers. Ten staff members, five men and Pive women,
collaborated in ibis work by reading the acoustic material. They were from 20
to 30 years old and had clear voices. Two lists were read by each speaker, the
first for learning and the second for recognition.
Vowels. Four French non-nasal vowels were chosen.
They were situated at the points and in the middle of the vowel triangle in the
F1F2 space.
Classic values for their formants are vowel F1 300 Hz
and F2 2,000 Hz; vowel /u/, F1300 Hz and F2 800 Hz; vowel F1
650 Hz and F2 1,250 Hz; and vowel /e/, F1 500 Hz and F2 1,500 Hz.
These vowels were well separated in the F1F2 space. Each vowel
was embedded in a sentence: "c'est /v/ ça," with /v/ standing for the
vowel. Each vowel was repeated 12 times at random to produce two 48-vowel
lists.
VOWEL RECOGNITION
Patient. Each patient was asked to recognize the vowels
spoken by each speaker. Confusion matrices were then estab-
Second
Dl C2 A2 Dl
C2E1 AIC1 Al E2 A2D2
C2E1E2 Cl DI D2 A2E1E2 C2D1D2
A2C1C2D2 AI A2C I D2 AIEIE2D1 AICIDID2
TABLE 2. FIRST AND SECOND BEST-MATCHING MODELS
Patient First
Single (I feature)
AM Cl
BA Cl
CO E2
LA D2
Pair (2 features)
AM ClE1
BA CID1
CO El E2
LA C1D1
Triplet (3 features)
AM CIE1E2
BA A2C2D2
CO A1E1E2
LA A2DID2
Quadruplet (4 features)
AM A2C1E1D2
BA A 1 A2E1E2
CO A 1A2EIE2
LA A2C1D1D2
lished. The patient first listened to the training list in
order to adapt his or her discriminating possibilitics. Then, for each
utterance of the recognition list, he or she was asked to give his or her best
choice for the vowel. A confusion rnatrix was constructed for each speaker and
for each patient, leading to a total of 40 matrices.
Computer. A previous study9 showed that
fuzzy logic, close to a probabilistic dccision, was well adapted to simulate
the patient's recognition of the vowels. Let us in troduce this method by
supposing that k features are studied for each vowel. The recognition process
can be broken into two stages. During the learning stage, a table is
filled out which records, for each value of the feature, the number of
occurrences corresponding to each class. Ranges have been normal ized from 1 to
32 for each item, and only integer values were taken. In each box of this
table, there is a histogram showing the occurrence of the 32 values.
This histogram was established in order to indicate the "probability" of each
of the 32 values. The CI mapping was adapted to each patient and a table
constructed for each implantce and each speaker. Consequently, a full
table contains 32 (values) x 4 (vowels) x 8 (features) = 1,024 numbcrs.
The features are dcscribed in the electrode number (E), the
amplitude (A), the duration (D), and the charge (C) (Fig 1). An example of the
most likely values, for each feature and for each vowel, is given in Table
1.
At the recognition stage, an "unknown" pattern "x"
needed to be classified. This pattern was represented by eight values (one for
each feature). For each feature, a score was attributed to each class. This
score was obtained from Table 1. The sum of the scores was calculated for each
vowel and x was classitied to the closest vowel (having the highest sum). When
all the vowels of the recognition list were classified, a confusion matrix was
established characterizing the automatic recognition.
Score of Model. A Hamming distance was constructed
between the confusion matrix observed with the patient, and the confusion
matrix of the automatic recognition (each automatic recognition corresponded to
a model). The sum of the absolute values of the difference, calculated box by
box between the two matrices, gave us the score of the model.
PARAMETERS
Signal Acquisition. The signal corning from the
speech processor was fed into a computer. In order to keep the same signal (for
the patients and for the automatic recognition), the lists were recorded on a
high-fidelity Revox tape recorder. The signal, transformed by the processor,
was taken by an acquisition card designed for this tank. The processor was set
according to the patient's map values. The system worked under the control of
the computer. Last, data were kept on disks (Fig 2).
It should be kept in mind that for each stimulating pulse,
representing a formant, the Nucleus device delivers six elementary
pulses bearing the information of the electrical stimulation. Out of these six
elementary-pulse sets, it is possible to extract the features of the
stimulating pulse (Fig 1): E, A,
D, and C. Positive and negative phases have the same
duration.
Set of Features. To facilitate the analytic study,
the Nucleus system was used according to the simple FOF1F2 strategy, and only
the information on the voice formants was considered. For each pitch period
during the utterance of a vowel, the Nucleus delivers two stimulating pulses
(one foreach formant) containing the following information (eight features): El
E2
A 1 A2 D 1 D2 Cl C2, with 1 and 2 referring to the pulse.
Thus, 255 recognition spaces can bc constructed with these features. Each space
(corresponding to a model) has a base that combines these eight features
according to the combinative analysis.
RESULTS
As the aim of the work is to cstimate which features arc
likely to bc used by CI subjects in the recognition process, a set of features
received a high score when its confusion matrix was close to the confusion
given by the patient ("bestmatching" model). Models were ranked according to
this proximity. Results are given in Table 2 averaging the 10 speakers. Thcy
are grouped according to the number of features.
DISCUSSION
Results showed that the recognition given by the models with a
single parameter did not always put the tonotopic information of the second
formant (E2) in top best-matching position. When two parameters were used, the
ElE2 combination was not systematically the bcst. Three times out of four, the
best-matching model was based on the first formant properties only (number and
energy).
When a third parameter was added, best-matching
models took information mostly from the two formants. It is worth noting that
thebest matching mode I changedfrom one patient to another. The settings of a
speech processor should take into account the patient's recognition strategy in
some way. This is now possible with the present CI versatility.
Schematically, we suggest the following interpretation of the
patients' results. For the star patient, CO, the results corresponded to the
tonotopic representation. For patient BA (prelingual deafness), the first
formant was mostly used, and the patient did not take full advantage of the
tonotopic representation. Patient LA, with only a small number of electrodes,
made excellent use of the information given by the charge. Patient AM (good
performer) had a tendency to take data from Fl and F2, which was not
specifically the electrode position.
It could be interesting in the future to generate the pulses
on a speech processor simulator, and to test directly, with the patients, the
best combinations given by the models.
CONCLUSION
This work considers, through the use of models, the importance
of some features of the stimulating pulse of a Nucleus speech
processor. This was done with CI patients using a corpus of four French vowels.
The main results can be summarized thus. The second formant position was not
always the best strategy for making the distinction. Data on the first formant
(including the charge) were also important. The classic phonetics model E1E2
was not always the bestmatching model. Again, data on the energy turncd out to
be equa]ly important. Relevant features differed from one patient to another,
suggesting that a strategy should be adapted to each subject. These results
need to be confirmed by testing in direct stimulation with the patients.
ACKNOWIF_DGME,;TS -- The authors thank people and
institutions that supporter/ thcir u ·ork: the Civil Hospitals of Lyon,
the French Council for Research, the Rhône-Alpes Region, the API company,
the University of Lyon, and Professor L. Collet from the Edouard Herriot
Hospital.
REFERENCES
1. Wilson BS, Finley CC, FarmerlC, Lawson DT. Comparative
studies of speech stratégies for cochlear implant. Laryngoscope
1988;98:1039-97.
2. Clark GM,Blamey PJ, Brown AM, et al. The University of
Melbourne Nucleus multi-electrode cochlear implant (monograph). Adv
Otorhinolaryngol 1987;38:1-190.
3. Belieff M, Dubus P, Leveau JM, Repetto JC, Vincent P.
Sound processing and stimulation coding of the Digisonic DX10 15-channel
cochlear implant. In: Hochmair-Desoyer IJ, Ilochmair ES, eds. Advances in
cochlear implants. Vienna, Austria: Manz, 1994:198-203.
4. Eddington DK. Speech recognition in deaf subjects with
multichannel intracochlear electrodes. Ann NY Acad Sci 1983;83:241-58.
5. Berger-Vachon C, Collet L, Djedou B, Morgon A. Model for
understanding the influence of some parameters in cochlear implantation. Ann
Otol Rhinol Laryngol 1992;101:42-5.
6. Dillier N, WaiKong L, Hans B. A high spectral transmission
coding strategy for multi-electrode cochlear implant. In: Hochmair-Desoyer II,
Hochmair ES, eds. Advances in cochlear implants. Vienna, Austria: Manz,
1994:152-7.
7. Doering WH, Schneider L. Electrical vs. acoustical speech
patterns of German phonemes using the Nucleus CI-system. In: Fraysse B, ed.
Cochlear implant, acquisition and controversies. Toulouse, France: Service ORL,
1989:243-53.
8. Blamey PJ, Clark GM. Place coding of vowels formants for
cochlear implant patients. J Acoust Soc Am 1990;88:667-73.
9. Gallego S, Perrin E, Berger-Vachon C, Collet L, Truy
E. Recognition of vowels by cochlear implant using a fuzzy logic, Int.
AMSE Modelling & Simulation Conference, Lyon, France, July 4-6, 1994. AMSE
Press, 1994;9:103-16.
15
9 iNI(8 )1131(7
|