6. Appendix
VaR.5% : -0.02851397 CVaR.5% : -0.04303770
Title:
MV Efficient Portfolio
Portfolio Weights:
Alsi Bank Beverage Chemical Equity.Inv Life.Insur
Telecom
0.0000 0.0854 0.1421 0.2242 0.0747 0.0000
0.0958
Technol USDZAR Risk.free
0.1244 0.2489 0.0044
Covariance Risk Budgets:
Alsi Bank Beverage Chemical Equity.Inv Life.Insur
Telecom
0.0000 0.1034 0.2012 0.2256 0.0814 0.0000
0.1432
Technol USDZAR Risk.free
0.2270 0.0185 -0.0003
Target Return and Risks:
mean mu Cov Sigma CVaR VaR
0.0024 0.0024 0.0171 0.0171 0.0372 0.0242
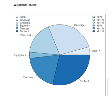
Iterations = 1001:11000
Thinning interval = 1
Number of chains = 1
Sample size per chain = 10000
1. Empirical mean and standard deviation for each variable,
plus standard error of the mean:
Mean SD Naive SE Time-series
SE
(Intercept) -0.0001138 8.713e-04 8.713e-06
1.073e-05
Dataset$Bank 0.1434273 3.481e-02 3.481e-04
2.628e-04
Dataset$Beverage 0.2692399 3.065e-02 3.065e-04
2.718e-04
Dataset$Chemical 0.1113228 3.898e-02 3.898e-04
3.467e-04
Dataset$Equity.Inv 0.0349513 3.327e-02 3.327e-04
3.460e-04
Dataset$Life.Insur 0.1670380 3.429e-02 3.429e-04
3.237e-04
Dataset$Telecom 0.1217420 2.772e-02 2.772e-04
2.699e-04
Dataset$Technol 0.1121895 2.673e-02 2.673e-04
2.366e-04
Dataset$USDZAR -0.0245311 3.799e-02 3.799e-04
3.799e-04
sigma2 0.0003003 2.175e-05 2.175e-07
2.134e-07
2. Quantiles for each variable:
2.5% 25% 50% 75%
97.5%
(Intercept) -0.0018314 -0.0007030 -0.0001028 0.0004741
0.0015800
Dataset$Bank 0.0758993 0.1192552 0.1433875 0.1667630
0.2121726
Dataset$Beverage 0.2088588 0.2486275 0.2690060 0.2898102
0.3288659
Dataset$Chemical 0.0353141 0.0851677 0.1112476 0.1371965
0.1893481
Dataset$Equity.Inv -0.0310190 0.0126328 0.0350995 0.0573648
0.1001137
Dataset$Life.Insur 0.0991277 0.1438379 0.1670621 0.1899787
0.2345747
Dataset$Telecom 0.0672014 0.1030527 0.1216117 0.1406669
0.1759571
Dataset$Technol 0.0593993 0.0945787 0.1124306 0.1299468
0.1643140
Dataset$USDZAR -0.0993244 -0.0497875 -0.0244516 0.0011008
0.0491126
sigma2 0.0002603 0.0002851 0.0002993 0.0003141
0.0003461
$priorPfolioWeights
a b c d e f
g
0.00000000 0.03770282 0.00000000 0.00000000 0.00000000 0.15294225
0.80935492
h
0.00000000
$postPfolioWeights
a b c d e f
g h
0.00000000 0.17475666 0.00000000 0.00000000 0.00000000 0.05346057
0.77178277
0.00000000
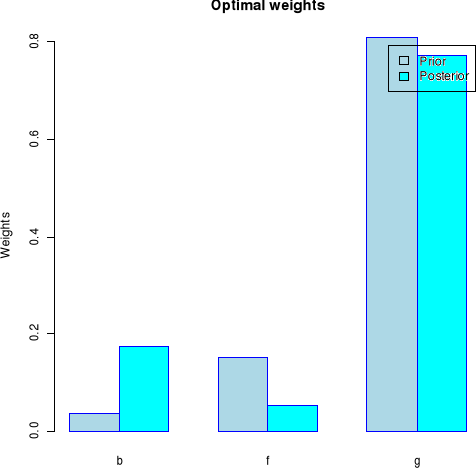
![]()
Title:
MV Tangency Portfolio
Estimator: .priorEstim
Solver: solveRquadprog
Optimize: minRisk
Constraints: LongOnly
Portfolio Weights:
a b c d e f g h
0.0417 0.2299 0.2235 0.0374 0.0000 0.1561 0.1818 0.1297
Covariance Risk Budgets:
a b c d e f g h
Target Return and Risks:
mean mu Cov Sigma CVaR VaR
0.0000 0.0030 0.0213 0.0000 0.0000
Description:
Tue Sep 28 15:35:54 2010 by user: General
$posteriorOptimPortfolio
Title:
MV Tangency Portfolio
Estimator: .posteriorEstim
Solver: solveRquadprog
Optimize: minRisk
Constraints: LongOnly
Portfolio Weights:
a b c d e f g h
0.0064 0.2508 0.2846 0.0000 0.0000 0.1357 0.1875 0.1350
Covariance Risk Budgets:
a b c d e f g h
Target Return and Risks:
mean mu Cov Sigma CVaR VaR
0.0000 0.0032 0.0214 0.0000 0.0000
Description:
Tue Sep 28 15:35:54 2010 by user: General
attr(,"class")
[1] "BLOptimPortfolios"
Iterations = 1001:11000
Thinning interval = 1
Number of chains = 1
Sample size per chain = 10000
1. Empirical mean and standard deviation for each variable,
plus standard error of the mean:
Mean SD Naive SE Time-series SE
(Intercept) 0.0009522 9.041e-04 9.041e-06 1.113e-05
Dataset$ebank 0.1398387 3.472e-02 3.472e-04 2.861e-04
Dataset$ebev 0.2688045 3.071e-02 3.071e-04 2.712e-04
Dataset$echem 0.1319496 3.712e-02 3.712e-04 3.282e-04
Dataset$eequit 0.0540071 3.231e-02 3.231e-04 3.318e-04
Dataset$elife 0.1775072 3.386e-02 3.386e-04 3.226e-04
Dataset$etelec 0.1266767 2.786e-02 2.786e-04 2.673e-04
Dataset$etech 0.1179304 2.654e-02 2.654e-04 2.457e-04
Dataset$eusazar -0.0094296 3.607e-02 3.607e-04 3.740e-04
sigma2 0.0003012 2.182e-05 2.182e-07 2.140e-07
2. Quantiles for each variable:
2.5% 25% 50% 75%
97.5%
(Intercept) -0.0008301 0.0003408 0.0009637 0.0015623
0.0027097
Dataset$ebank 0.0720157 0.1161651 0.1402517 0.1631226
0.2073024
Dataset$ebev 0.2085894 0.2482177 0.2686163 0.2893781
0.3291133
Dataset$echem 0.0599178 0.1070750 0.1317707 0.1565901
0.2057048
Dataset$eequit -0.0100727 0.0322763 0.0539325 0.0757838
0.1172233
Dataset$elife 0.1106212 0.1548548 0.1774808 0.2002120
0.2440648
Dataset$etelec 0.0719587 0.1081133 0.1265930 0.1455601
0.1808797
Dataset$etech 0.0657202 0.1002334 0.1182699 0.1358541
0.1696935
Dataset$eusazar -0.0808365 -0.0333380 -0.0095017 0.0147699
0.0617244
sigma2 0.0002611 0.0002860 0.0003002 0.0003151
0.0003471
|